In today’s industrial landscape, driven by Industry 4.0 and advancements in artificial intelligence in manufacturing, maintaining optimal machine performance is crucial for operational efficiency and staying competitive.
By leveraging predictive maintenance software, businesses can analyze equipment data to detect potential issues before they escalate. This proactive approach utilizes specialized sensors and computing systems to monitor machinery, providing early warnings of possible failures.
Addressing problems in advance reduces downtime, enhances equipment longevity, and prevents costly repairs, leading to smoother operations.
This guide delves into predictive maintenance, exploring its mechanisms, distinctions from preventive maintenance, the role of AI, and real-world applications.
- What is predictive maintenance?
- How does predictive maintenance work?
- What is the difference between predictive and preventive maintenance?
- How is AI used in predictive maintenance?
- What are some examples of predictive maintenance technologies?
- What are some predictive maintenance use cases?
- A future outlook on predictive maintenance
- People Also Ask
What is predictive maintenance?
In software, predictive maintenance refers to the proactive use of data analytics, AI, and machine learning to monitor system performance and predict potential issues before they disrupt operations.
It involves continuously analyzing logs, usage patterns, and performance metrics to identify anomalies that could signal impending failures. By anticipating these issues, teams can implement corrective actions, minimizing downtime and optimizing software reliability and performance.
Predictive maintenance in software helps extend system life, enhances user experience, and reduces costs associated with emergency fixes or reactive maintenance.
How does predictive maintenance work?
Predictive maintenance uses technology to keep equipment running smoothly now and in the future. It gathers data from IoT sensors, controls, and other business systems connected to machinery. This data may include heat, sound, pressure, and vibration readings.
Data goes to the cloud, usually into an AI-powered IT asset management (ITAM) or computerized maintenance management system (CMMS). ML processes the data to predict equipment health and identify problem areas.
Real-time analysis helps maintenance teams respond quickly. They can fix issues as soon as they arise or even before.
AI-driven forecasting also improves related processes. ML models predict when to do maintenance, schedule services, and manage supplies, supporting “just-in-time” maintenance and reducing unexpected downtime.
Here’s an overview of the key technologies that underpin predictive maintenance:
- IoT sensors: Sensors on equipment collect data like temperature, pressure, and vibration. This real-time info shows how machines run and can catch early signs of problems.
- Cloud transformation: Cloud systems store and organize data from sensors. This setup allows quick, remote access to information, making it easy to track equipment health.
- AI and ML: AI reviews data to spot patterns and predict maintenance needs. ML identifies trends over time, allowing teams to fix issues before they grow and reducing unexpected downtime.
What is the difference between predictive and preventive maintenance?
Both predictive and preventive maintenance help keep equipment working well, but they work differently.
Predictive maintenance uses AI to spot problems before they happen. It watches equipment in real-time and uses current and past data to predict future issues. This means teams can fix things only when needed, which saves time and money.
Preventive maintenance follows set schedules and checklists. It uses basic software to plan maintenance dates and follows standard steps to fix issues. While this works for basic needs, it can’t adjust quickly to sudden changes like predictive maintenance can.
Let’s explore how these two methods differ below:
Feature | Predictive Maintenance | Preventive Maintenance |
Data Analysis | Uses AI and ML to check equipment in real-time | Uses set schedules and standard processes |
Monitoring | Shows how systems are running at all times | Uses basic checklists and manuals |
Problem-solving | Spots and fixes problems before failures | Follows fixed maintenance dates |
Data focus | Looks at current and past equipment data | Based on past equipment performance |
Predictive capability | Can predict future equipment problems | Can’t adjust to sudden changes |
Maintenance timing | Fixes issues only when needed | Fixes things on a set schedule |
Cost-effectiveness | Cuts down on system failures and costs | May fix things that don’t need fixing |
Adaptability | Adjusts to changing equipment needs | Not good for changing operations |
Response time | Responds right away to equipment issues | Waits for scheduled maintenance dates |
Proactive approach | Suggests fixes before problems happen | Follows the same plan regardless of need |
How is AI used in predictive maintenance?
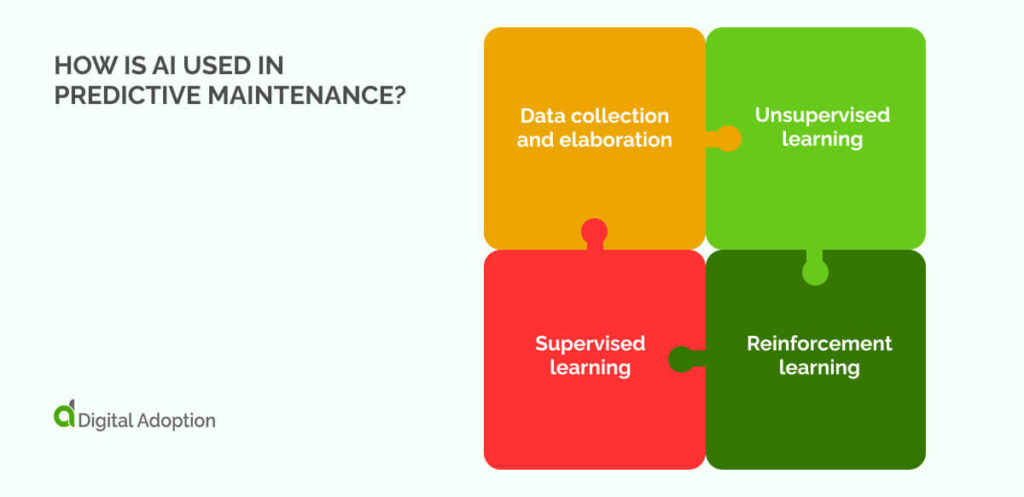
AI and ML are now augmenting functions typically done by maintenance teams. It is freeing up human productivity and reaching capabilities once thought impossible.
Let’s explore exactly how AI is used in predictive maintenance:
Data collection and elaboration
AI needs a lot of data to work well in predictive maintenance. It gets this data from equipment, IoT sensors, and integrated systems.
These touchpoints constantly observe how machines work. ML algorithms also examine records of past performance and problems, creating a strong foundation of information about how equipment should normally work.
Unsupervised learning
AI uses unsupervised learning to find hidden patterns in abstract equipment data. This technique is special because AI learns without being told what to look for. It’s great at finding unusual behavior that might mean trouble.
For example, unsupervised learning can spot these changes if a machine starts making different vibrations or using more power than usual. This helps catch new problems that haven’t happened before.
Supervised learning
With supervised learning, AI learns from examples of past equipment failures. It studies data from times when things went wrong before, helping AI understand specific signs that trouble is coming.
It teaches AI to recognize warning signs and can warn maintenance teams early if these patterns appear again. The more examples AI sees, the better it gets at predicting similar problems.
Reinforcement learning
This is where AI gets smarter over time. Reinforcement learning helps AI improve its predictions by learning from what works and what doesn’t. When AI makes a good prediction about maintenance needs, it remembers what worked.
When it misses something, it adjusts its approach. This helps create better maintenance schedules that get more accurate over time. The system keeps learning and adapting as it sees more data and results.
What are some examples of predictive maintenance technologies?
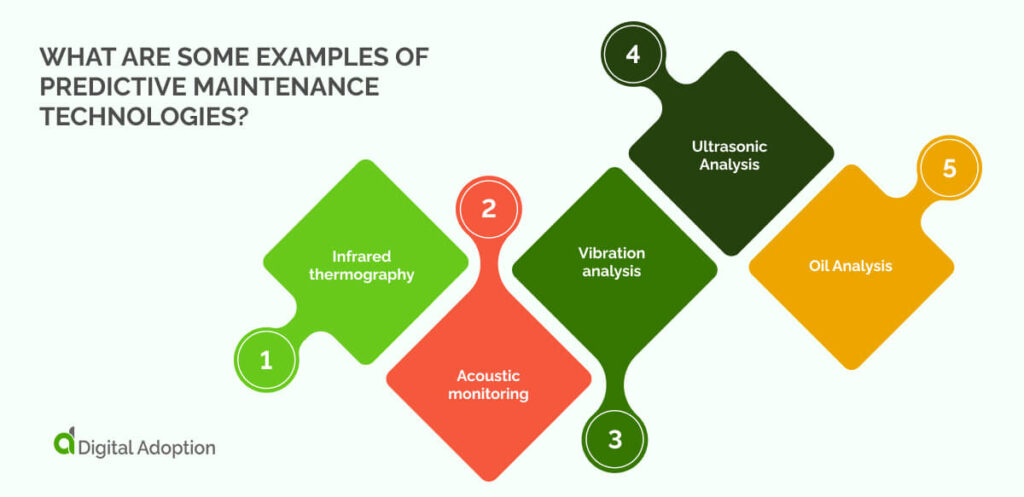
Predictive maintenance is needed to monitor and manage a wide range of equipment.
In manufacturing, critical machines use different technologies to track temperature, sound, and other factors.
Let’s look at some key technologies used in predictive maintenance:
Infrared thermography
Infrared thermography uses special cameras to spot heat in machines. Every machine generates heat when it works. If a machine is too hot, it might have a problem. For example, an overheating motor could fail soon.
Technicians can use this technology to find heat issues quickly, helping them make repairs before problems arise. Infrared thermography is especially useful for checking electrical parts, motors, and bearings. It helps keep equipment running smoothly.
Acoustic monitoring
Acoustic monitoring listens to the sounds that machines make while they operate. Each machine has its own sound. If a machine starts making unusual noises, it could indicate trouble.
For instance, a strange grinding sound might mean a bearing is failing. Sensors can pick up these sounds and analyze them. This early detection allows technicians to fix issues before they lead to costly repairs. Acoustic monitoring is a smart way to maintain machines and avoid downtime.
Vibration analysis
Vibration analysis checks the vibrations produced by machines. All machines vibrate, but excessive or unusual vibrations can signal problems. For example, if a pump vibrates too much, it might be misaligned or have worn parts.
Technicians use sensors to track these vibrations over time. This data helps them see patterns that indicate potential failures. Using vibration analysis, companies can schedule repairs before serious breakdowns occur, helping keep operations running smoothly.
Ultrasonic Analysis
Ultrasonic analysis uses high-frequency sound waves to find problems in equipment. It is great for detecting leaks in systems that use compressed air or steam.
This technology picks up sounds that humans cannot hear. When there is a leak, it creates an ultrasonic sound. Technicians can find these leaks early, which helps save energy and reduce costs. This method is an effective way to improve equipment efficiency and prevent waste.
Oil Analysis
Oil analysis tests the lubricant used in machines. It provides important information about the machine’s health. By examining oil samples, technicians can find metal particles or other contaminants.
These findings can show wear or possible failures. For example, a high metal level in the oil might indicate that a bearing is wearing out. Regular oil analysis allows maintenance teams to plan repairs based on the machine’s condition. This approach extends the equipment’s life and reduces unexpected breakdowns.
What are some predictive maintenance use cases?
Businesses need to understand how they can predict when machines will need fixing.
Looking at how other companies do this, they can see the benefits, such as having their machines break down less often and spending less on repairs.
When businesses know these things, they can make better choices about caring for their equipment in ways that work best for them.
Let’s explore some predictive maintenance use cases:
Predictive maintenance in transportation and logistics
Predictive maintenance helps keep vehicles and equipment working safely in transportation and shipping. Special sensors watch how vehicles run and warn workers about problems like engine trouble, worn-out brakes, or bad tires.
Shipping companies use this information to fix delivery trucks before they break down and cause late deliveries. Airlines also use it to check their plane engines and find problems early, making flights safer and preventing cancellations.
Trains can prevent accidents by spotting rail and wheel problems quickly. Keeping vehicles healthy and fixing them at the right time ensures everything runs smoothly and packages arrive on time.
Predictive maintenance in manufacturing equipment
The manufacturing sector is undergoing a digital transformation, and predictive maintenance is at its heart.
Predictive maintenance is changing how factories work by keeping machines running better and stopping surprise breakdowns. Special sensors that detect shaking can tell when motors aren’t working right, warning workers early.
For example, Ford uses this technology in its car factories to keep assembly lines running smoothly. Computer chip makers monitor machine temperatures to prevent overheating, which could damage parts.
Watching how machines run in real time lets factories plan repairs around their work schedule, causing fewer disruptions. This helps machines last longer and cost less to maintain, assisting factories in making better products.
Predictive maintenance in smart cities
Smart cities use predictive maintenance to keep everything working safely and efficiently. For example, sensors on bridges measure how strong they are and if they’re rusting so city workers can fix them before they become unsafe.
Buses and trains have special technology that tells repair teams when something’s wrong, helping prevent service delays. Garbage trucks can also have sensors to improve routes and reduce pollution. Smart streetlights can predict when bulbs will burn out, keeping streets lit and safe.
Through predictive maintenance, smart cities stay safer, use resources more efficiently, and provide better services to residents.
Predictive maintenance in energy infrastructure
Power companies use predictive maintenance to keep electricity flowing and prevent blackouts. Sensors in power plants watch for unusual movements in turbines, finding problems before they become major failures.
Power companies check how transformers are working to prevent overheating and power outages. Wind farms use predictive maintenance to check their blades, planning repairs to keep them spinning. In oil and gas, pipeline sensors detect pressure changes to catch leaks or rust early.
Predictive maintenance helps preserve important equipment, keep power flowing, save money on repairs, and keep workers safe.
Predictive maintenance in defense
Predictive maintenance helps keep military equipment ready for action and working properly. Military planes use smart technology to check engines and systems, keeping aircraft flying and cutting repair costs.
Military vehicles use data to determine when parts need replacing so repairs can be made before missions. Navy ships track how well their engines and radar work to stay safe at sea.
Predictive maintenance in the military keeps soldiers safe, maintains important equipment, and helps forces stay ready for action by stopping unexpected breakdowns.
A future outlook on predictive maintenance
Predictive maintenance is a key method for helping businesses with quality assurance and asset management. It enables critical business infrastructure to work better and is crucial for lowering operational costs and increasing IT efficiency.
In the future, businesses in sectors such as transportation, manufacturing, energy, and defense will increasingly rely on predictive maintenance powered by AI.
For example, shipping companies may use AI-driven drones equipped with sensors to monitor the condition of cargo ships in the real world. These drones would analyze data on vessel performance, weather conditions, and potential obstacles, allowing operators to make proactive decisions about maintenance needs before problems surface.
As predictive maintenance improves, businesses can expect smarter ways to keep their machines running longer and better.
People Also Ask
-
Can predictive maintenance be applied to different industries?Yes. Smart machines that spot problems early can help all kinds of businesses. This includes everything from factories making items to trucks delivering packages. These machines watch how the equipment works and warn people before something breaks, which helps keep everyone safe and everything running smoothly.
-
How does predictive maintenance contribute to cost reduction?Predictive maintenance helps save money by stopping machines from breaking down unexpectedly and reducing the time they are out of service. Fixing problems before they worsen means businesses spend less on repairs and make their machines last longer. This also helps utilize resources better and plan maintenance more effectively.
-
How can predictive maintenance be integrated into existing enterprise systems?Businesses can add IoT sensors to their machines that connect to computers. These sensors watch how the machines work and send information to a main computer system. This helps workers know exactly when something needs fixing without changing everything about their work.