Large language models (LLMs) are one sect of AI gaining momentum for their natural language processing and understanding capabilities. Generative AI platforms like ChatGPT, Midjourney AI, and Claude leverage LLMs to generate a wide array of content via text-based inputs.
One technique that makes these platforms more effective is generated knowledge prompting, which stands out for its ability to enhance AI’s reasoning and output quality. This technique enables LLMs to build on their existing knowledge, leading to more dynamic and context-aware interactions.
This article will explore generated knowledge prompting. We’ll explore how it works and look at some examples before diving into some practical applications to help you understand its potential and implement it effectively in your AI-driven projects.
- What is generated knowledge prompting?
- How does generated knowledge prompting work?
- What are some examples of generated knowledge prompting?
- Applications of generated knowledge prompting
- Generated knowledge prompting vs. traditional prompting vs. chain-of-thought prompting
- Pushing boundaries with generated knowledge prompting
- People Also Ask
What is generated knowledge prompting?
Generated knowledge prompting is a prompt engineering technique where AI models build on their previous outputs to enhance understanding and generate more accurate results.
It involves LLMs reusing outputs from existing knowledge into new inputs, creating a cycle of continuous learning and improvement.
This helps the model develop better reasoning, learning from past outputs to give more logical results. Users can use one or two prompts to make the LLM generate information. The model then uses this knowledge in later inputs to form a final answer.
Generated knowledge prompting tests how well LLMs can use new knowledge to improve their reasoning. It helps engineers see what LLMs can and can’t do, revealing their limits and potential.
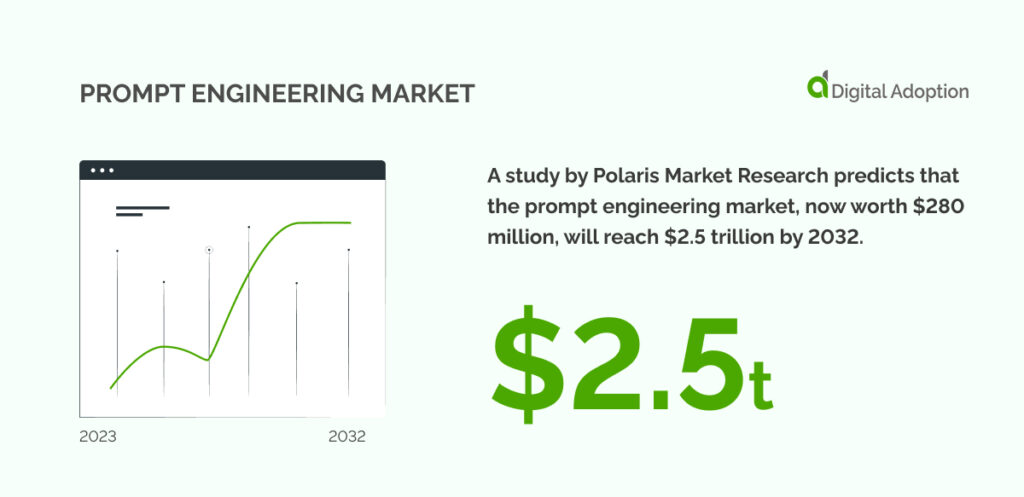
A study by Polaris Market Research predicts that the prompt engineering market, now worth $280 million, will reach $2.5 trillion by 2032. It’s growing at 31.6% yearly due to more AI chats, voice tools, and the need for better digital interactions.
How does generated knowledge prompting work?
When working with large language models (LLMs), text prompts guide the model to produce targeted content based on its training data. This capability becomes especially useful when users need to generate specific insights or trends.
For example, a sales leader might request insights on recent sales trends by prompting the LLM with, “Identify key B2B software sales trends from the past five years.” The model would then generate a list of patterns, including customer preferences and emerging technologies.
These insights serve as a foundation for further analysis. Once the trends are outlined, sales managers can review and refine the results to ensure they align with real-world conditions.
This makes it easier to integrate the findings into strategies, such as comparing quarterly performance to identified trends: “Compare our Q3 sales data with these trends and highlight areas for improvement.”
The model can then identify gaps or missed opportunities in performance, guiding decision-making for future strategies.
What are some examples of generated knowledge prompting?
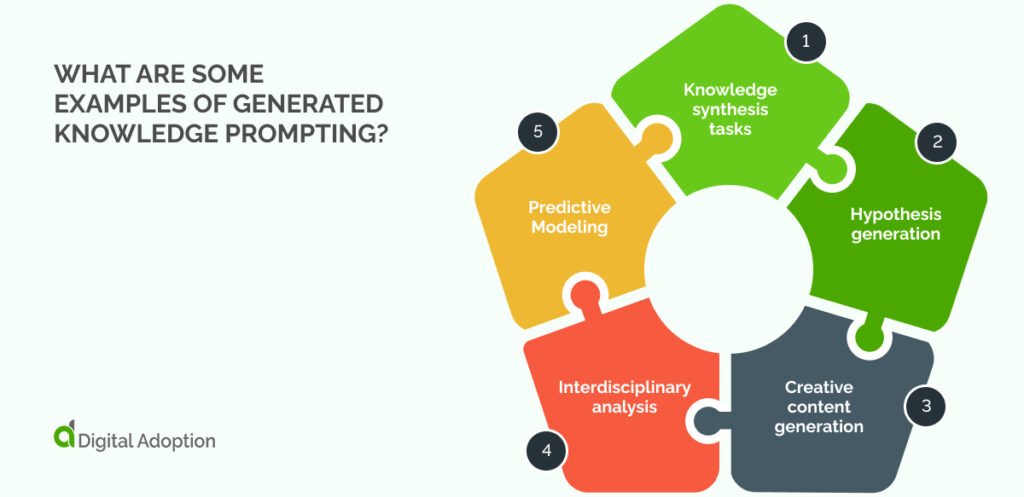
This section provides practical examples of applying generated knowledge prompting across different tasks.
The desired results can also be achieved by prompting the model to generate knowledge and integrate it into the following outputs in a single request. This is known as the single prompt-generated knowledge approach.
These examples follow a dual prompt-generated knowledge approach. Each example includes a prompt and a clear structure to show how knowledge is generated and integrated into further steps.
Knowledge synthesis tasks
The vast pools of data LLMs are trained on, and their natural language understanding (NLU) and natural language processing (NLP) functions mean they can efficiently process complex information to create a clear, cohesive summary.
Step 1: Knowledge generation
Prompt: “Summarize key trends in artificial intelligence (AI) development over the past decade.”
LLM Output:
1. Growth of machine learning models
2. Rise of natural language processing (NLP)
3. Increased focus on AI ethics
Step 2: Input refinement (Knowledge integration)
Prompt: “Based on these trends, suggest how AI might evolve in the next five years.”
LLM Output:
1. Expansion of AI in healthcare
2. Enhanced AI governance frameworks
3. Greater integration of AI in everyday consumer tech
Hypothesis generation
When it comes to building research (verifiable) and hypotheses, generated knowledge prompting can provide new ideas from diverse sources.
Here’s an example:
Step 1: Knowledge generation
Prompt: “List key factors contributing to global warming based on recent studies.”
LLM Output:
1. Increased CO2 emissions
2. Deforestation
3. Fossil fuel consumption
Step 2: Hypothesis creation (Knowledge integration)
Prompt: “Using these factors, suggest hypotheses on how global warming affects marine ecosystems.”
LLM Output:
1. “If CO2 emissions rise, ocean acidification will increase, disrupting marine life.”
2. “Deforestation impacts ocean carbon absorption, leading to warmer sea temperatures.”
Creative content generation
For generating creative content, this method helps generate new ideas by recycling and repurposing the model’s pre-existing knowledge.
Step 1: Knowledge Generation
Prompt: “Provide a summary of key themes in classic science fiction literature.”
LLM Output:
1. Space exploration
2. Human-robot relationships
3. Time travel and parallel worlds
Step 2: Creative content generation (Knowledge integration)
Prompt: “Write an opening paragraph for a science fiction story about human-robot relationships.”
LLM Output:
“In the year 2100, robots weren’t just machines—they were companions, confidants. But when a group of androids started exhibiting human emotions, society was forced to confront the blurry line between technology and humanity.“
Interdisciplinary analysis
For complex topics that involve multiple disciplines, generated knowledge prompting can connect the dots.
Step 1: Knowledge generation
Prompt: “Explain the role of AI in modern biology, particularly in drug discovery.”
LLM Output:
1. AI algorithms identify drug candidates faster
2. Machine learning aids in predicting drug efficacy
3. AI enhances patient-specific treatment development
Step 2: Interdisciplinary insight
Prompt: “Analyze how AI can improve the efficiency of drug discovery processes.”
LLM Output:
“AI reduces the time needed for molecule identification and testing, making drug discovery faster and more cost-effective. This is particularly useful for developing personalized treatments based on genetic data.“
Predictive Modeling
The crucial data underpinning predictive models can be enhanced through generated knowledge prompting. The information generated by LLMs can enrich the predictive models’ existing data and expand its knowledge pool.
More detailed forecasts gleaned through AI-assisted back-knowledge allow for more accurate predictions in complex scenarios.
Step 1: Knowledge generation
Prompt: “Summarize recent trends in stock market performance for the technology sector.”
LLM Output:
1. Steady growth of tech stocks
2. Increasing investments in AI and cloud technologies
3. Volatility due to global supply chain issues
Step 2: Predictive Modeling
Prompt: “Based on these trends, predict how tech stocks may perform in the next year.”
LLM Output:
“Tech stocks will likely continue to grow, driven by advancements in AI and cloud services. However, ongoing supply chain disruptions could cause short-term volatility.“
Applications of generated knowledge prompting
Generated knowledge prompting has diverse real-world applications. Understanding these is important because they demonstrate how AI can augment human capabilities and drive innovation across sectors.
Let’s take a closer look:
Enhanced research capabilities
Generated knowledge prompting can reshape how research is done. Students and scholars worldwide have long used tried-and-true methods for finding and studying information.
This technique lets researchers go deeper than surface-level analysis. Feeding data from prior prompts into the model boosts its grasp of a topic.
Once trained, the model can see the big picture, spotting complex links in the transformed data. This way, researchers can do advanced studies that tap into new trends while improving research quality and quantity.
Innovation and ideation
Generated knowledge prompting offers a structured way to create ideas. The process often starts with prompts that push AI to explore broad areas.
For example, a first prompt like “Suggest new materials for eco-friendly packaging” sets the stage for brainstorming.”
More specific prompts can then guide the AI to certain industries or limits, such as, “Focus on materials that cut carbon footprints by 30% or more” or “Propose cost-effective and durable solutions.”
By layering prompts that narrow the focus, AI can create new solutions that meet specific business or technical needs. The ability to generate winning ideas faster than old methods has sparked digital innovation across many fields.
Scientific discovery support
Testing ideas and boosting research are key to scientific discovery.
Generated knowledge prompting can aid these processes, refining knowledge for better results.
Researchers often start with a broad question, like “Find potential treatments for Alzheimer’s,” and use the AI’s answer as a starting point.”
With each new prompt, the questions get more specific, maybe focusing on one protein or pathway, like, “Review new studies on tau protein’s role in brain diseases.”
This guides the model to give more precise answers, helping researchers build a solid framework for tests.
A good template prompt could be, “Look at current gene therapy trial data and suggest new areas to explore.“
Advanced problem-solving
For complex issues, generated knowledge prompting breaks the problem into smaller parts, guiding AI through a layered analysis.
The process starts with broad prompts like, “Identify main causes of global supply chain problems.”
The AI finds key factors and later prompts us to investigate each one—maybe focusing on “How changing fuel prices affect shipping delays” and then “Suggest new routes to reduce these delays.”
This step-by-step approach lets AI tackle complex problems, offering solutions based on data and deep analysis.
Scenario analysis and forecasting
Scenario analysis and forecasting greatly benefit from generated knowledge prompting by structuring prompts that explore future possibilities.
For instance, a first prompt might ask, “Predict the economic effects of a 10% global oil price rise over five years.”
Follow-up prompts can refine the AI’s response. Examples include “Analyze how this price hike would impact Southeast Asian markets” or “Suggest ways for vulnerable industries to cope with this change.”
This detailed, step-by-step prompting helps AI forecast multiple scenarios, giving businesses nuanced insights into possible futures.
Generated knowledge prompting vs. traditional prompting vs. chain-of-thought prompting
Generated knowledge prompting elevates AI interactions by guiding the model through iterative, context-enriching prompts.
It is different from traditional and chain-of-thought prompting.
Let’s look at how:
Generated knowledge prompting
Generated knowledge prompting enhances AI interactions through iterative, context-rich prompts. Each new input builds on previous AI responses, deepening understanding and revealing insights. This method allows for advanced, nuanced exploration of complex topics, especially in research and innovation.
Traditional prompting
Traditional prompting uses one-off, isolated queries. The AI gives single, static answers based only on the current input. While quick for simple tasks, it lacks depth and continuity for complex analysis or problem-solving.
Chain-of-thought prompting
Chain-of-thought prompting falls between the other two. It uses a logical sequence of prompts to guide AI through step-by-step reasoning. Each prompt helps the AI break tasks into smaller, manageable parts. While good for complex problems, it doesn’t let the model build broader understanding like generated knowledge prompting does.
Pushing boundaries with generated knowledge prompting
Generated knowledge prompting is one method that aims to reach new levels of depth and precision in AI systems.
Whether in science, business strategy, or forecasting, this technique marks big steps in how these fields research, innovate, and solve problems.
Using prompt engineering wisely will be key to developing ethical AI. As AI use grows across industries, it will handle more critical tasks where accuracy is vital.
Poorly designed prompts can increase risks, potentially harming the success of AI projects.
Ensuring data integrity and reliable, verifiable inputs is crucial for maintaining the quality and trust in large language models (LLMs) outputs.
People Also Ask
-
What are the advantages of generated knowledge prompting?Generated knowledge prompting can help bring many advantages to modern LLM capabilities. Reinforcing model learning through this method allows for more sophisticated and nuanced outputs. Key advantages include: Enables AI to build a deeper, contextual understanding Enhances research capabilities through iterative refinement Excels in complex problem-solving and innovation Uncovers overlooked patterns and connections
-
What are the limitations of generated knowledge prompting?While powerful, generated knowledge prompting does come with certain challenges and limitations. These constraints are important to consider when implementing generated knowledge. Limitations include: Requires careful and precise prompt engineering Time-consuming and resource-intensive Risk of compounding errors if early prompts are misguided Effectiveness depends on the users skill in managing the process Generated knowledge may not achieve the desired depth without well-structured prompts and is generally more intricate than traditional prompting methods.
-
What is a dual prompt approach?The dual prompt approach is a sophisticated method that enhances AIs analytical capabilities. It combines two types of prompts in a strategic manner. Heres a breakdown: 1. The first prompt generates broad, high-level insights. 2. The second prompt then narrows the focus for deeper analysis or specific details. This effectively balances scale and scope, delivering both comprehensive exploration and focused recommendations. Its strength lies in conducting complex queries that require both macro-level overview and micro-level examination.