Ongoing tech advancements have sparked feverish digital transformations in the field of manufacturing.
Out of this new digital era emerges Industry 4.0, where innovative tech solutions aim to transform manufacturing and production processes globally.
Cloud adoption, blockchain, AR and VR, and AI are just some examples of technologies whose impacts are being felt industry-wide.
Key developments driving the fourth industrial revolution cannot go without mentioning AI. The ability to teach computers to learn from data and self-improve creates efficient AI solutions. This is helping to fast-track core processes, smart manufacturing capabilities, and supply-chain transformation.
Today’s top business executives understand that capturing AI’s value is key. Doing so may mean the difference between becoming future-ready and becoming irrelevant.
This article will explore how to implement AI in manufacturing. We’ll delve into what it is, why it matters, and how its role today plays a part in the success of tomorrow’s businesses.
What is AI in manufacturing?
In manufacturing and production environments, it involves swapping out traditional processes for the adoption and integration of AI-powered technologies.
Key technologies like machine learning (ML), deep learning (DL), and computer vision (CV) underpin modern AI. In manufacturing, the data generated across vast IoT networks and smart industrial systems fuels these techniques.
These models learn from data to recognize patterns, inform decisions, and predict future trends. AI prevails in predicting system maintenance needs. Pattern detection allows engineers to preempt disruptions and minimize system downtime. ML models can also monitor inventory levels and forecast demand. Automation capabilities like these lead to reduced waste and better resource allocation.
AI also enhances robotics and automation, enabling smart machines to augment human capabilities. AI-driven robots can confidently perform intricate tasks with precision, limiting the need for human interference. These smart machines benefit from learning from their environment and self-improvement.
The accuracy and speed of AI are enhancing efficiency and productivity and paving the way for smarter, more adaptive manufacturing processes.
Why does AI in manufacturing matter?
Technology’s role in manufacturing is integral to ensuring business viability and success in the modern age. Today, major AI developments only reaffirm technology’s role in creating new value for businesses worldwide.
AI is revolutionizing manufacturing by streamlining processes and enhancing operational efficiency. One key advancement is predictive maintenance, where AI anticipates equipment failures before they occur, significantly reducing downtime and minimizing disruptions.
AI also enables real-time data analysis, which provides manufacturers with actionable insights for informed decision-making. This leads to improved quality control and a faster response to changing market conditions. These AI-driven improvements optimize production workflows, boost efficiency, and drive innovation. They ensure manufacturing operations are more agile and resilient.
This trend looks set to continue with the realization of Industry 4.0. AI will become a major influencer in the next industrial revolution by further advancing smart manufacturing systems.
As industries embrace AI, they will benefit from more agile and adaptive production environments, which are crucial for maintaining a competitive edge in an uncertain future.
Methods for implementing AI in manufacturing
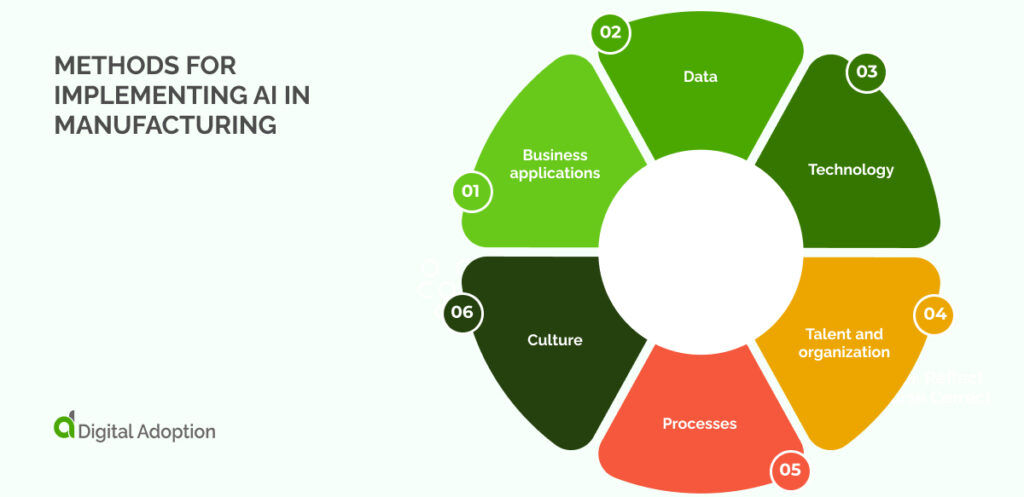
Manufacturing companies illustrate that combining technological investments and strategic organizational shifts is crucial to excelling in large-scale AI adoption.
This section delves into the core business, technical, and organizational adjustments required for effective AI implementation.
These adjustments are organized into six distinct categories:
Business applications
Manufacturers must identify where new technologies can make the most impact to build a successful strategy. This involves evaluating potential applications across different parts of the business and organizing them by their likely benefits and the effort required to implement them.
Prioritizing these applications helps identify key projects that can serve as examples of success, driving broader adoption and laying the groundwork for future technological and organizational changes.
Data
Effective technology use relies heavily on robust data management. Manufacturers must create systems that centralize and integrate information from various sources. This includes production sensors and operational systems.
Establishing comprehensive data repositories helps create a complete picture of operations. Incorporating external data sources further enhances this picture. Proper data organization is essential for accuracy and accessibility. Quality control and tracking are crucial for supporting better decision-making and operational efficiency.
Technology
As technology evolves, companies must carefully plan their digital infrastructure. Developing a clear framework for the tools and systems needed to handle, process, and analyze data is crucial. Using established, widely accepted technologies for managing large datasets and performing complex analyses can provide scalable and effective solutions.
Tools that handle continuous data streams from production equipment should receive special attention to ensure they meet the needs of the manufacturing environment.
Talent and organization
Building a capable team is vital for effectively leveraging new technologies. Manufacturers should assemble diverse specialists with expertise in data handling, engineering, and system design. A central team can lead the development and deployment of new solutions.
This team works closely with various departments to tailor applications to specific needs. Over time, these roles may become more integrated within different parts of the organization. This integration creates a cohesive network of expertise.
Processes
Streamlined operations depend on well-defined processes and governance. Companies should establish clear procedures for evaluating and implementing new solutions, ensuring that all stages, from initial assessment to deployment, are managed efficiently.
Proper data governance and adherence to best practices and ethical standards are essential for maintaining smooth operations and scaling solutions effectively.
Culture
Creating a supportive environment is key to the successful adoption of new technologies. Companies need to build confidence in these technologies by educating employees about their benefits and limitations. Addressing concerns about job impacts and emphasizing the advantages of collaborative work between people and technology can help encourage acceptance.
Leadership should actively support and promote these changes, prompting experimentation and celebrating successes to drive widespread adoption throughout the organization.
How is AI transforming the manufacturing industry?
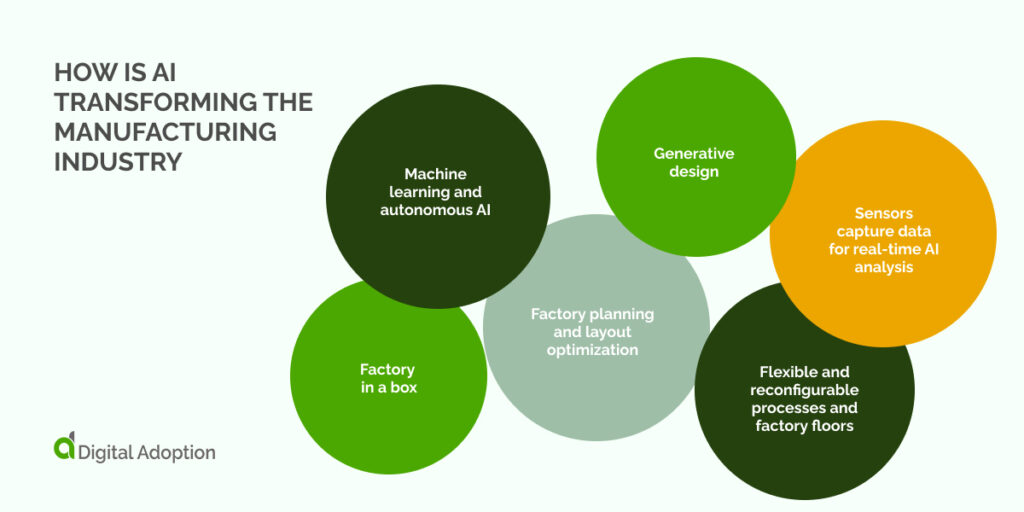
At the heart of this evolution is the quest for operational efficiency. This is where AI technologies streamline processes and reduce waste, significantly enhancing productivity.
AI advancements contribute to better agility and quality. They allow manufacturers to adapt to changing demands through precision and innovation.
Let’s take a look at how AI is transforming the manufacturing industry:
Factory in a box
Factory in a Box represents a significant leap in manufacturing efficiency and scalability. This innovation consolidates all essential components into a single modular unit. It includes machinery, control systems, and processes. This consolidation minimizes setup time and maximizes space utilization.
Integrating advanced manufacturing technologies within this compact system allows for rapid deployment. It also provides adaptability to diverse production needs. Manufacturers can quickly adjust to market demands and scale operations without extensive infrastructure.
This approach reduces capital expenditure. It also enhances operational flexibility, achieving high production standards in a streamlined, cost-effective manner.
Machine learning and autonomous AI
Machine learning and autonomous AI transform manufacturing by enabling systems to process vast amounts of data. They make informed decisions with minimal human oversight.
These technologies optimize production processes through continuous learning and adaptation. This improves operational efficiency and product quality. Machine learning algorithms analyze both historical and real-time data. They identify patterns, predict potential issues, and enhance decision-making.
Autonomous AI systems independently execute tasks such as quality control and maintenance scheduling. This reduces downtime and operational costs. Integrating these technologies creates a more responsive and intelligent manufacturing environment. It drives innovation and competitiveness in the industry.
Factory planning and layout optimization
Factory planning and layout optimization are pivotal in enhancing manufacturing efficiency. Manufacturers can significantly reduce production time and operational costs by meticulously designing the arrangement of equipment, workstations, and workflow processes. Advanced planning tools enable the simulation of various layout scenarios. These tools also allow for the analysis of different configurations. This process helps businesses identify the most efficient arrangements.
This strategic approach minimizes material handling, reduces bottlenecks, and improves overall process flow. The result is a streamlined manufacturing environment that maximizes productivity and resource utilization.
Effective layout optimization also supports flexibility, making it easier to adapt to changes in production demands and technological advancements.
Sensors capture data for real-time AI analysis
Sensor deployment in manufacturing systems is crucial for capturing real-time data for AI analysis. These sensors monitor various parameters, including machine performance, environmental conditions, and product quality. They generate a continuous stream of actionable information.
AI algorithms process this data to detect anomalies and predict maintenance needs. They also optimize process parameters. Real-time analysis allows for immediate responses to potential issues. This reduces downtime and enhances overall operational efficiency.
Manufacturers can use sensor data to achieve higher precision. It also improves quality control and maintains a proactive approach to equipment management and process optimization.
Generative design
Generative design reshapes product development using computational algorithms to explore many design alternatives. This process involves defining design parameters and constraints, after which the system generates numerous potential solutions that meet the specified criteria.
The resulting designs are often more efficient and innovative compared to traditional approaches. Generative design facilitates the creation of optimized, lightweight, and cost-effective components by considering factors such as material usage, strength requirements, and manufacturing methods.
This capability accelerates the design phase, reduces material waste, and fosters the development of high-performance products aligned with precise engineering standards.
Flexible and reconfigurable processes and factory floors
Flexible and reconfigurable processes and factory floors enable manufacturers to adapt to evolving production needs and product variations. This adaptability is achieved through modular systems and dynamic process configurations that can be easily adjusted or reconfigured.
Such flexibility allows for changes in production volume, product type, and process requirements. These adjustments occur without significant downtime or capital investment. Reconfigurable factory floors also facilitate efficient space utilization. They streamline workflows, enhancing the ability to respond to market shifts and customer demands.
This approach supports a more agile manufacturing environment, driving innovation and operational resilience.
What does the future hold for AI in manufacturing?
AI in manufacturing promises transformative advancements and reshapes industry standards and operational strategies.
Businesses that integrate AI will likely experience substantial benefits. These include enhanced productivity, reduced operational costs, and improved product quality. Those that adopt AI-driven predictive maintenance, real-time analytics, and automation will gain a competitive edge through optimized processes and reduced downtime.
Integrating advanced technologies will also facilitate more agile responses to market fluctuations. This will allow businesses to adapt swiftly to evolving customer demands and trends.
Manufacturing companies should invest in AI technologies to capitalize on these advancements. This includes adopting scalable AI solutions, promoting a culture of continuous learning, and developing strategies to integrate AI into existing operations.
Businesses can use AI to drive growth, enhance efficiency, and maintain a strong position by preparing for these changes.