Key processes that once required ample time and human resources to complete are now being augmented through artificial intelligence (AI).
As organizations realize its many benefits, AI has become viable across all business levels in nearly every industry. These systems emulate the cognitive capabilities of humans to detect patterns, make key decisions, and automate back-office actions.
The AI adoption race, however, brings with it the real risks of AI bias.
Organizations must recognize how AI bias can arise if solutions aren’t properly vetted and steps aren’t taken to mitigate its negative impact.
This article explores five real-world AI bias examples. From GenAI missteps to biased hiring algorithms at Amazon, enterprises can learn from these stories to ensure strong AI safety practices support their digital transformation efforts.
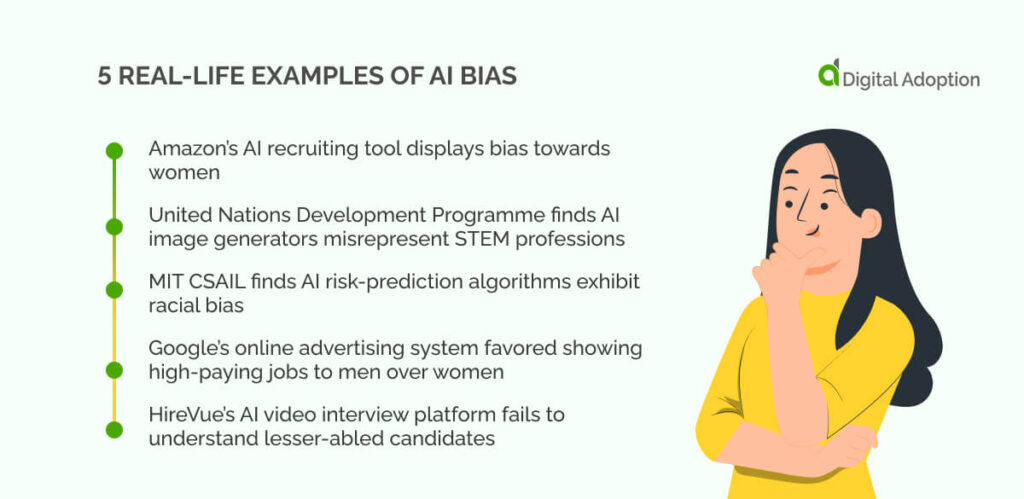
- 1. Amazon’s AI recruiting tool displays bias towards women
- 2. United Nations Development Programme finds AI image generators misrepresent STEM professions
- 3. MIT CSAIL finds AI risk-prediction algorithms exhibit racial bias
- 4. Google’s online advertising system favored showing high-paying jobs to men over women
- HireVue’s AI video interview platform fails to understand lesser-abled candidates
- Can AI ever be free of bias?
- People Also Ask
1. Amazon’s AI recruiting tool displays bias towards women
Amazon’s flawed automated AI recruiting tool displayed bias towards female job candidates.
The eCommerce giant deployed the experimental AI recruiting tool using a five-star rating system that scored their likelihood of getting software and technical job roles at the company.
The pattern detection capabilities of Amazon’s recruitment tool AI were trained to spot similarities across applicant resumes. To achieve this, they had been analyzing countless applications for up to a decade.
With those typically assuming technical and software positions in the past being disproportionately men, Amazon’s AI algorithm began to skew reasoning toward these preferences.
The AI began showing signs of sexism, lowering scores for resumes from women and steering its preferences toward male candidates. Applicants who had attended one or more all-female universities were also ranked lower.
Even after re-training the system to act in a gender-neutral way, Amazon shut down the project once it became clear the AI still made unfair judgments.
According to Statista, candidate matching was the most common use of AI in North American recruiting.
With a 60% share of the current global workforce, it’s likely men will be overly represented in data used to train AI. For enterprises embracing AI, mitigating bias is pivotal to ensuring solutions are fair and ethical for all.
2. United Nations Development Programme finds AI image generators misrepresent STEM professions
Generative AI (GenAI) applications have given rise to diverse forms of AI. Underpinning GenAI are large language models (LLMs) that communicate and understand users through natural language capabilities.
LLMs have helped fuel the popularity of image generators like Midjourney and DALL-E, which artificially create detailed and stylized images in minutes.
While exploring gender gaps in STEM professions, researchers at the United Nations Development Programme’s (UNDP) Accelerator Lab assessed how two popular AI image generators, DALL-E 2 and Stable Diffusion, perceived women in STEM professions.
When prompted to generate visual images of the words “IT expert,” “mathematician,” “scientist,” or “engineer,” both DALL-E 2 and Stable Diffusion reflected societal stereotypes, illustrating that men naturally fit STEM positions over others.
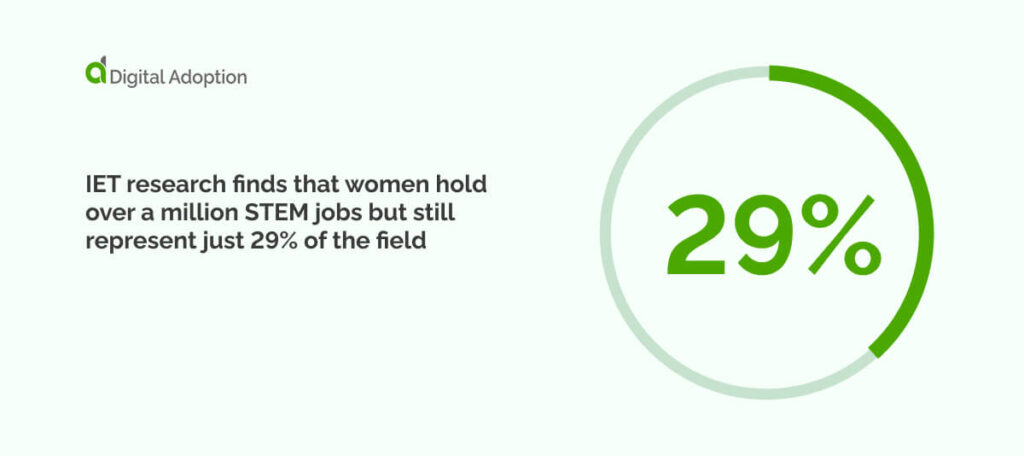
IET research finds that women hold over a million STEM jobs but still represent just 29% of the field. AI can replicate real-world gender gaps in fields where women are often left out if the training data contains human bias—whether clear or hidden—and can produce unfair results toward certain groups.
If AI systems aren’t checked properly or the data isn’t cleaned of bias, it can cause ethical problems in hiring, promotions, inclusion, and learning or development chances for minority groups.
3. MIT CSAIL finds AI risk-prediction algorithms exhibit racial bias
A study published by researchers at MIT’s Computer Science and Artificial Intelligence Laboratory (CSAIL) in collaboration with MIT found that AI risk prediction algorithms were shown to exhibit racial bias.
Over 900 people took part in a test to study how AI might shape decision-making. They were shown parts of a fake crisis hotline script involving men in a mental health emergency. Each script also included details like race and religion—such as whether the man was African American, white, or a practicing Muslim.
The AI would make either prescriptive or descriptive recommendations based on what it learned from the call. Prescriptive advice is more direct and calls for explicit actions such as calling the police, whereas descriptive provides more ambiguous recommendations and summaries of events.
AI models trained on old or biased data—like racial prejudice or skewed historical records—can end up suggesting police action for African Americans or Muslims more than others.
In one experiment, people who followed biased AI advice during urgent tasks made more discriminatory choices. That’s risky, especially when the AI influences users who wouldn’t normally act with bias.
When those same people made decisions without AI input, their choices didn’t show bias tied to race or religion.
4. Google’s online advertising system favored showing high-paying jobs to men over women
Researchers at Carnegie Mellon University launched an auto-testing tool that showed how online recommendation engines such as Google Ads display gender bias. Dubbed AdFisher, the tool deployed over 17 thousand fake profiles simulating male and female jobseekers.
The profiles were exposed to over half a million job adverts, and researchers were able to run rigorous browser-based experiments that assessed for bias.
Findings show that high-paying coaching jobs offering over $200k annual salary were advertised 1,852 times to men and only 318 times to women. Generic ads, such as an auto-dealer position, were recommended to the female profiles.
Looking closely at Google’s ad settings matters, especially because of its size and global influence. Google uses browsing history, online activity, cookies, and personal info to decide who sees which ads—while giving users some control. But the way AI uses this data can affect which groups are targeted, and differences in targeting don’t always mean bias.
In hiring, diversity, equity, and inclusion (DEI) are often seen as core to progress. So, when companies use online AI tools, they need to promote privileged positions fairly and not exhibit unexplained preferential treatment to certain groups.
HireVue’s AI video interview platform fails to understand lesser-abled candidates
AI-powered interview platforms with bias present can disadvantage candidates with disabilities, leading to hiring bias and legal risks for enterprises.
An example of this is HireVue’s AI video interview tool, which failed to interpret the spoken responses of lesser-abled candidates applying for jobs. The platform’s AI captured these responses to generate transcripts of online interviews.
When a deaf and Indigenous woman applied for a role at the software firm Intuit, the automated speech recognition system failed to understand or interpret her responses.
She has a deaf English accent and communicates using American Sign Language (ASL). The model had no training or mechanism to process these inputs accurately.
Bias in recruiting tools like HireVue’s often mirrors real-world views that overlook minority behaviors—such as deafness, non-standard speech patterns, or dialects like African American Vernacular English (AAVE). Voices from older adults, people of color, or those with disabilities are often misread or dismissed because of this.
The candidate requested human-generated captions to follow interview questions and instructions but was denied. Intuit later rejected her for promotion. In its feedback, the company told her to “build expertise” in “effective communication,” “practice active listening,” and “focus on projecting confidence…”
With AI now embedded in enterprise systems, biased recruiting tools can quietly turn exclusion into standard practice. For enterprises, this means adopting practices that rigorously test AI systems for accessibility gaps before they automate discrimination at scale.
Can AI ever be free of bias?
Whether AI can fully be free of bias depends on the integrity of training and the data behind it.
Today, enterprises often choose third-party AI-driven solutions instead of building in-house tools from scratch. Even with strong frameworks, AI risks can be built into the system before deployment, making it easy to miss risks at every stage—training, data collection, processing, and labeling.
Enterprises can take steps to keep their solutions risk-free. They can invest in digital tools to test for biases and regularly audit AI models for fairness. Having diverse teams oversee data input and model training is also important.
While AI may never be completely free of bias, businesses can reduce dangers by being proactive and transparent. This helps create inclusive systems that avoid discrimination, ensuring AI benefits the wider society as a whole.
People Also Ask
-
Is GenAI bias?Yes, GenAI can be biased. It learns from data that might have biases or stereotypes. If the data is biased, the AI can repeat those biases in its answers or actions.
-
What can enterprises do to mitigate AI bias?Enterprises can try new methods like using synthetic data to balance out biased real-world data. They can also test AI systems to see how they handle bias and fix any issues. Explainable AI (XAI) helps by showing how decisions are made, making it easier to find and fix biases.
-
What methods help mitigate AI bias?Enterprises rely on three methods to control AI bias. AI governance sets rules to ensure that systems are fair and accountable. It also shapes how AI is built and used by applying clear ethical standards. Data governance manages the quality and fairness of training data. It checks how data is collected and maintained to avoid bias at the source. AI development frameworks provide structure for building fair systems. They help teams catch and correct bias early in the design process.