Business transformation is becoming increasingly intertwined with AI adoption.
However, if not approached carefully, the rush to become the first movers in the AI race can carry some very real risks. One danger that today’s firms need to be aware of is AI bias.
AI bias occurs when machine learning (ML) algorithms or the data they’re trained on are skewed or embedded with human and societal biases. Developers’ assumptions can unknowingly become ingrained in AI coding, leading to potentially discriminatory outputs.
Businesses must mitigate bias in AI. Failure to do so can erode digital trust, harm reputations, and breach legal regulations.
This article will explore AI bias and why alleviating it should be a top business priority. We’ll also examine some leading principles, the different types of AI bias, and how they impact modern industry.
What is AI bias?
AI bias is when human and societal biases and prejudices are absorbed by machine learning algorithms and the data used to train AI systems.
This can happen when AI developers’ existing biases and preconceptions mistakenly enter AI design during coding. It can also occur in the training data AI relies on to understand and contextualize the real world.
Improper checks during data collection can lead to skewed training data with social imbalances and inequalities. ML algorithms leverage this data to perform actions and make key decisions. If undetected, bias in the foundational training of AI models can greatly undermine how they interpret new data.
This can create a snowball effect, where a small bias in training data undermines the basis for future learning and model reasoning. AI systems generate potentially harmful outcomes, which can reinforce and amplify negative stereotypes and discriminatory behavior.
Why is eliminating bias from AI important?
Preexisting societal biases and generalizations are a fact. However, major ethical concerns arise when these imbalances become systemized into the technology used by society and business.
AI’s rise has seen it adopted at nearly every level, from governments to businesses. Its huge applicability means it is used in HR hiring processes, analyzing credit scores, conducting financial audits, and supporting law enforcement.
AI already impacts people’s lives in the real world, making mitigating AI bias even more important. Baked-in AI bias can disproportionately affect marginalized groups, such as women, people of color, or those with limited mobility or a particular economic standing.
Enterprise-grade AI is expected to be accurate, reliable, and explainable. Should missteps in AI reflect biases towards marginalized groups or perform business operations based on false or incomplete data, it can not only ruin public trust but lead to reputational harm or hefty fines for failing to meet compliance.
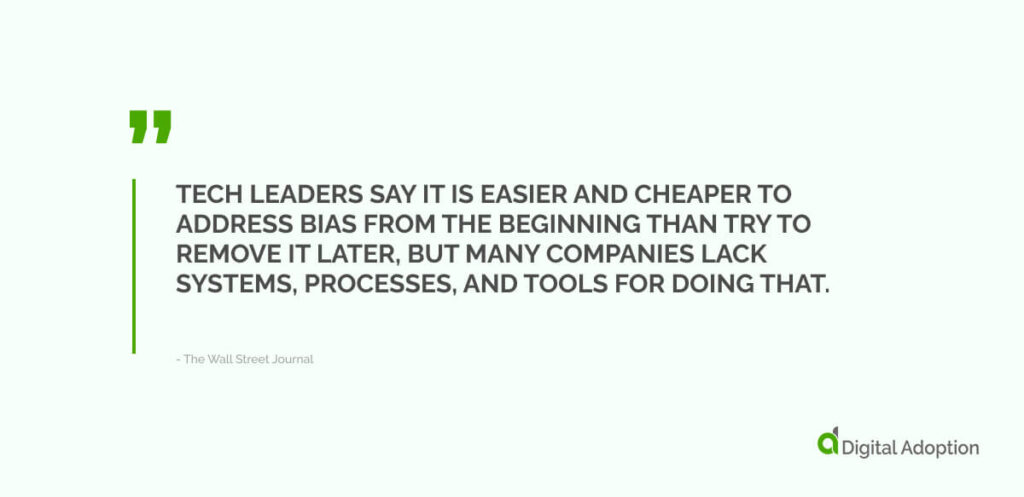
How does AI bias affect different industries?
AI’s wide applicability means it’s finding a place industry-wide. However, this seemingly unlimited potential has drawbacks, including equal ways to derail business operations. These risks are too high to ignore in industries like healthcare and finance.
Let’s explore a few industries where AI has demonstrated bias:
Healthcare
AI bias in medical diagnosis distorts treatment decisions, especially for underrepresented groups. Predictive models rely on historical data, which often excludes diverse patient populations.
As a result, conditions like heart disease may go undiagnosed in women, while pain management recommendations skew toward certain racial groups. Automated triage tools further reinforce these disparities, misclassifying symptoms based on incomplete or skewed datasets.
When AI-driven systems misinterpret risk factors, patients may receive delayed or inadequate care, deepening existing inequities in AI healthcare access and outcomes.
Human Resources
Hiring algorithms inherit bias from past recruitment patterns, filtering out qualified candidates based on race, gender, or socioeconomic background.
AI-powered applicant tracking systems often favor candidates whose resumes resemble those of past hires, unintentionally sidelining those from nontraditional backgrounds. Automated performance evaluations can deepen workplace inequities, as HR AI interprets productivity metrics without accounting for structural biases in assignments or opportunities.
When unchecked, these systems create hiring cycles that reinforce existing inequalities, limit workforce diversity, and perpetuate unfair advantages.
eCommerce
AI bias shapes customer interactions, from product recommendations to pricing. Algorithms trained on incomplete or skewed data prioritize certain demographics, influencing who sees high-value products or exclusive deals.
Chatbots trained on biased sentiment analysis misinterpret dialects, leading to inconsistent or dismissive responses. Even dynamic pricing systems can reflect discriminatory patterns, adjusting costs based on data correlations that disadvantage specific groups.
These biases, embedded in AI-driven finance, affect purchasing experiences and reinforce unequal access to products and services.
What are the most common types of AI bias?
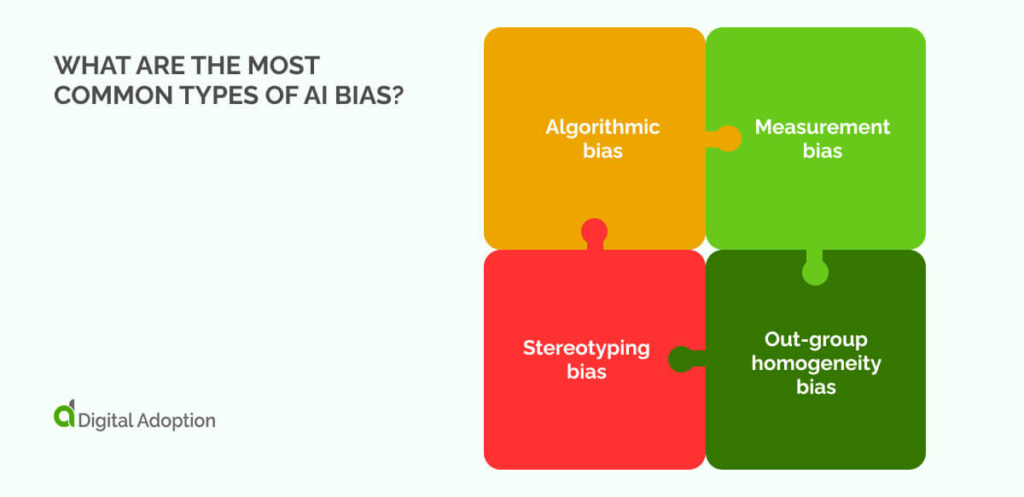
AI bias comes in two forms: explicit and implicit. Implicit bias is harder to spot. It appears when AI learns from past data that reflects human prejudices, carrying them forward in ways people don’t always notice.
Below, we look at a few types of implicit bias and how they can appear in AI:
Algorithmic bias
AI follows patterns, but those patterns aren’t always fair. For example, hiring systems trained on past resumes might favor candidates who fit a certain mold, ignoring equally qualified people with different backgrounds. Predictive policing tools direct officers to certain areas based on past crime data, even when that data reflects over-policing rather than actual crime. Loan systems also reject applicants based on biased financial histories, limiting opportunities for some groups.
Measurement bias
AI makes mistakes when its training data doesn’t match real life. For example, facial recognition struggles with accuracy when trained mostly on white faces, leading to misidentifications of people with darker skin. Medical AI also misdiagnoses conditions in women, as it was trained mostly on male data. When AI relies on incomplete data, it reinforces gaps rather than solves them.
Stereotyping bias
AI learns stereotypes from the data it is trained on. For example, hiring tools might assume men are better for certain jobs and reject qualified women before anyone sees their applications. AI chatbots can repeat harmful ideas from the internet, and image recognition software may wrongly label jobs based on old-fashioned beliefs about gender.
Out-group homogeneity bias
AI often treats different people as if they are all the same, ignoring their unique traits. It may misunderstand the way people speak, assuming everyone from a place talks the same. Recommendation systems may show content similar to that of entire groups, limiting choices and shaping what people see unfairly.
What are some principles for mitigating AI bias?
Bias enters AI systems through data, design, and decision-making processes. While AI bias cannot be removed entirely, organizations can reduce its impact by following structured principles.
These principles are listed below:
AI governance
AI systems need clear rules for development, testing, and deployment. Organizations should create policies that set strict guidelines for data selection, model training, and performance monitoring. Regular audits will also help identify bias before AI is used in real-world settings.
Developers should document decisions at every stage and ensure models follow ethical and legal standards, such as the EU AI Act. Without strong governance, AI can reinforce harmful patterns without anyone noticing.
Fairness
AI should work equally well for all groups. Training data must represent different demographics, preventing the system from favoring one over another. Developers should run bias tests, measuring how AI performs across race, gender, age, and other factors.
If the system shows unfair results, adjustments should be made before deployment. Fair AI does not mean treating everyone equally. It means ensuring outcomes are not skewed against certain groups.
Transparency
Users and regulators need to understand how AI makes decisions. Companies should disclose what data AI models use, how they are trained, and what risks they carry. Black-box systems, where AI operates without explanation, create trust issues. Open documentation and clear communication prevent AI from becoming an unchecked force that reinforces hidden biases.
Human oversight
AI should not operate without human review. Automated systems make mistakes, especially when handling complex or sensitive tasks. People must monitor AI decisions, checking for biased patterns and correcting errors when needed. Regular testing ensures AI remains fair as data and conditions change. Without human oversight, AI bias can go unnoticed and cause long-term harm.
What are some best practices for avoiding bias in AI?
To reduce bias, organizations must apply structured practices throughout AI development.
Below are some ways these can be used when mitigating bias in solutions:
Algorithmic fairness assessment
AI models should be tested for fairness before deployment. Companies must assess how algorithms perform across different groups, checking for favoritism or harm. Regular fairness assessments help spot issues early and make adjustments.
Select appropriate training data and model
AI learns from the data it’s trained on. Using diverse, representative data reduces bias, ensuring the model doesn’t perpetuate harmful stereotypes or ignore certain groups.
Perform thorough data processing
Data must be cleaned and structured correctly. Incomplete or unbalanced data can lead to biased AI outcomes. Companies should remove irrelevant or duplicated data to improve model accuracy.
Introduce human-in-the-loop systems
Humans should oversee AI decisions. Automated systems can miss context, and human input ensures biases are spotted and corrected.
Embed fairness into algorithms
Fairness should be built into the algorithms themselves. Developers must create models that adjust for bias during the training process.
Will AI ever be fully unbiased?
From intuitive GenAI chatbots that understand and converse with customers to predictive maintenance that detects and prevents equipment failures, AI is helping enterprises become future-ready.
But can AI ever be completely fair? That depends on how well people control its development.
Bias may never fully disappear, but it can be reduced. Careful testing, better training data, and ongoing human oversight help catch unfair patterns before they cause harm. Diverse teams bring different perspectives, making AI more balanced.
Companies must continue improving their models, checking for bias, and fixing problems as they arise. With the right approach, AI can become as fair as possible, but it will always need human guidance.
People Also Ask
-
Is AI bias worse than human bias?AI bias can be more harmful because it spreads faster and affects more people at once. Humans can recognize their biases and try to correct them, but AI follows patterns in data without questioning them. If left unchecked, AI can repeat and even increase unfair patterns, making them harder to detect and fix.
-
How often does AI bias occur?AI bias is common, especially in systems trained on old or unbalanced data. Since AI learns from records, it often repeats the same mistakes. Bias appears in hiring, healthcare, and law enforcement, where AI can favor certain groups based on flawed data.
-
What role does diversity play in addressing AI bias?Diverse teams help reduce bias by spotting problems that others might miss. When AI is trained with data from different backgrounds and perspectives, it makes fairer decisions. The more varied the experiences used in training AI, the less likely it is to favor one group over another.