The time of big data has arrived. Businesses know they need to collect customer activity data to stay competitive. Data can empower a company to increase workforce optimization, improve change management processes, and provide a better customer experience.
But the advantage given by data isn’t free— it takes work to get the most out of data while avoiding the regulatory pitfalls. Digital transformation must happen for companies to truly use data to their advantage. Companies need the right frameworks, policies, and tools to maximize their data’s value.
Digital transformation starts with understanding customer needs. Companies must ensure that their customers’ data is collected and utilized ethically and securely.
That’s where Data Lifecycle Management (DLM) comes in.
Data Lifecycle Management is businesses’ most widely used approach to handling data.
Praised for its simplicity, Data Lifecycle Management makes it easy to collect, store, use, and dispose of data. It helps businesses mitigate the risks associated with carrying data and ultimately leads to a better understanding of what it takes to leverage data successfully.
We’ve put together this guide on everything you need about Data Lifecycle Management. Armed with this knowledge, we’ll prepare you to take your business to the next level and tap into the benefits of data.
- What Is Data Lifecycle Management (DLM)?
- Understanding The Importance of Data Lifecycle Management
- The Three Primary Objectives Of Data Lifecycle Management
- The Five Stages Of The Data Management Lifecycle
- How Can Data Lifecycle Management Help SMEs?
- What is Hot, Warm, and Cold Data?
- The Benefits Of Having A Set Process For Managing Data
- Data Lifecycle Management Best Practices
- Data Lifecycle Management Alternatives
- Looking Ahead: What’s Next For Data Lifecycle Management?
What Is Data Lifecycle Management (DLM)?
The given definition for DLM is “the application of a set of principles, processes, and technologies for data management and storage.”
But what does that mean?
Put simply, DLM helps businesses get the most out of data. It’s a comprehensive set of practices that not only help enterprises to capture better data but also ensures they’re handling that data correctly.
DLM covers many essential data management areas, including data governance and security, storage, archiving and disposal, backup/recovery, scalability/performance tuning, analytics, and reporting. It also includes several additional practices that can help optimize how businesses handle their data.
DLM aims to create a more efficient and cost-effective data storage system that allows businesses to get the most out of their data. DLM helps organizations develop faster, more accurate business insights, reduce operational costs, and support agile decision-making.
Understanding The Importance of Data Lifecycle Management
In an increasingly data-driven world, large amounts of data are unwieldy and difficult to manage. Without DLM, a data scientist looking for the proper subset of data is like looking for a needle in a haystack, but all of the hay is just numbers and letters on a screen.
DLM helps ensure the right data is in the right place at the right time. This makes it easier for data scientists or analysts to draw meaningful, relevant, and timely conclusions. It helps analysts generate more value for businesses more quickly.
Understanding The Chief Data & Analytics Officer Roles In Future Enterprises is critical to implementing an effective DLM process. A CDO is responsible for delivering data and analytics solutions and services that enable organizations to achieve business objectives through data assets.
Furthermore, if data is valuable to a business, it benefits competitors and malefactors. Companies must protect data. They must handle all data in adherence to internal and external regulations— the consequences for failing to do so are dire.
Data lifecycle management streamlines the process of protecting and managing data.
Neglecting to leverage data lifecycle management is a dangerous game with little return.
The Three Primary Objectives Of Data Lifecycle Management
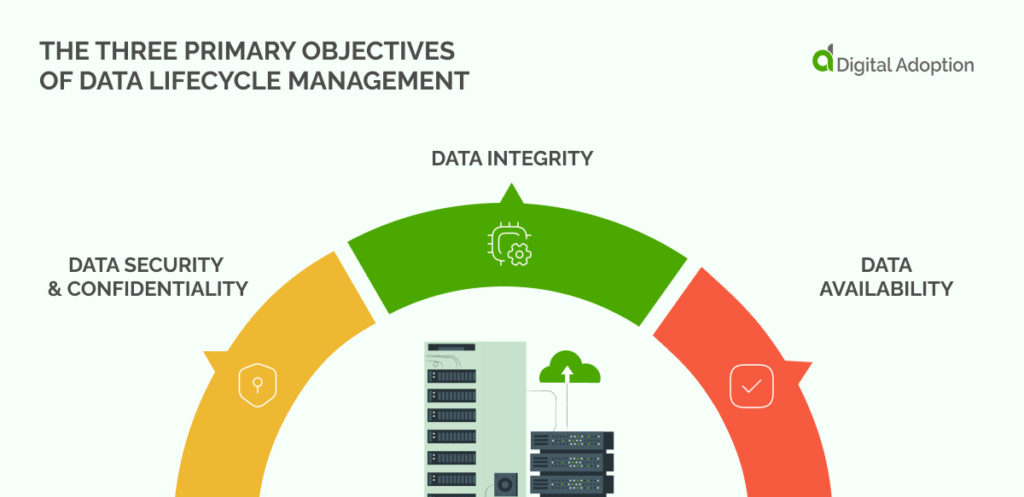
- Data Security & Confidentiality
For human-focused companies, data often comes from information about people, whether customers or employees.
Employee or customer data is often personal and sensitive. Collecting it requires permission, which comes with a responsibility to protect the data from unauthorized access. That’s why data security should be a top priority for businesses.
DLM provides guidance on how to collect data legally and share data securely. The framework has been developed with data protection policies at the core.
It helps maintain confidentiality by ensuring businesses delete data thoroughly.
- Data Integrity
Data is only useful if you trust it, which is why data maintenance is critical to businesses. When using data to inform major business decisions, you must be able to count on the data being reliable, precise, and accurate.
Poor data integrity can be dangerous, leading you to draw incorrect conclusions with confidence.
DLM helps you maintain the integrity of any collected data by outlining how that data should be handled, copied, and stored.
- Data Availability
Data by itself does nothing. Teams of people need to analyze and manipulate it. While one objective of DLM is to protect data from those who shouldn’t be able to access it, another objective is to keep it readily available to those who can access it.
Data lifecycle management aims to ensure the teams who need to access data can, exactly when they need to, with minimal friction.
Notably, DLM also aims to make data archiving more effective. By following processes for data archival, businesses are less likely to delete data and regret it because they need it later.
The Five Stages Of The Data Management Lifecycle
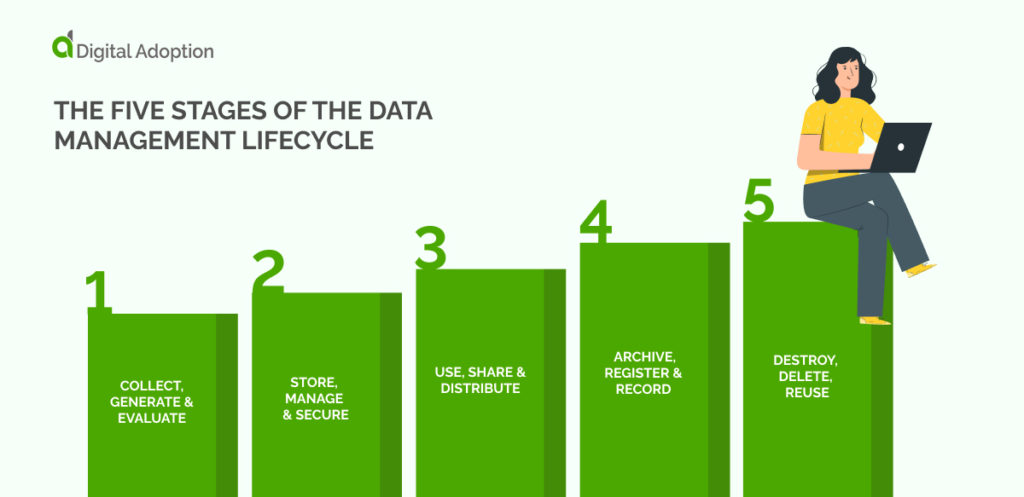
According to the data lifecycle management framework, these five stages are designed to break down the data life cycle into manageable chunks.
They’re written to apply to all businesses, which means you might have additional business rules for data processing, which you may want to implement at the appropriate stage.
1. Collect, Generate & Evaluate
Data collection methods are varied and abundant.
Enterprise applications, IT infrastructure, and internet of things (IoT) devices are just a few examples of quantitative data collection.
Surveys, feedback forms, and opinion polls are examples of qualitative data collection.
The general idea with data creation is “more is better.” But don’t be afraid to use intuition to judge whether a particular data creation initiative wastes resources.
After data collection comes data classification, where you should evaluate data for potential uses and classify it so that it’s easier to find and refer to later.
If you gather data but believe it won’t be helpful, you should discard it at this stage— it’s an early opportunity to carry out some data cleaning.
2. Store, Manage & Secure
Once collected, you need to store data somewhere. But the best solution for storing data depends on the kind of data.
Quantitative data is usually considered “structured data.” Cold hard metrics, numbers, and objective values are relational. That means it’s easy to relate and compare to other relational data sets.
It’s common to store such data in a relational database system.
Conversely, qualitative data is usually “unstructured data.” It’s text-heavy and not relational, so storing it in a non-relational database (like NoSQL) is more common.
Data protection is also critical at this stage. You should encrypt sensitive data for the sake of data protection. Data storage is a tricky business, and there are governmental policies in place, like GDPR, which mandate good data protection practices from companies.
Now that you’ve protected the data from external threats, you must also protect it from internal threats. Backing data up provides redundancy, which makes it trivial to recover should any data-loss event occur.
3. Use, Share & Distribute
Outages aren’t the only internal threat to data. This stage of DLM defines who within an organization can access data and what they can use it for.
Typically, data undergoes a process called “data munging” before it’s usable for humans. This is transforming or manipulating data to make it relevant and readable.
Generally, this is the time when data handling is most sensitive. You should have audit trails of who accessed which data and when. You should only give access to sensitive data if there’s sound business justification.
In some cases, companies use data collected by external companies, especially for marketing and advertising purposes.
4. Archive, Register & Record
Once data has outlived its usefulness, you should archive it. This is an in-between stage, where a business doesn’t think it needs the data but wants to retain the possibility of accessing it just in case.
Archive locations are usually less accessible than live data storage environments. This makes for more efficient storage.
However, archived data should be accessible without needing IT to restore it from a backup storage device. It’s essential to getting the most out of your data and could be necessary for investigative purposes.
Archived data is typically stored in separate storage space from operational data. The data migration process from the live environment to the archive is a change request.
5. Destroy, Delete, Reuse
It can be tempting for an organization to skip this step because “the more data, the better, right?”
Wrong.
You absolutely cannot ignore the data destruction stage. As regulations continue to develop, it’s an increasingly important step.
It’s important to understand that stored data you don’t need is a waste of resources and exposes you to unnecessary risk.
Most big businesses have a data retention policy that dictates how long they keep static data. Once the time outlined in this policy has passed, you must perform data deletion.
Some regulations, like GDPR, mandate data deletion under the right circumstances. GDPR states that all citizens have a “right to be forgotten,” so a business must delete their data following a written request from a citizen.
So it’s essential to have a process for data deletion.
How Can Data Lifecycle Management Help SMEs?
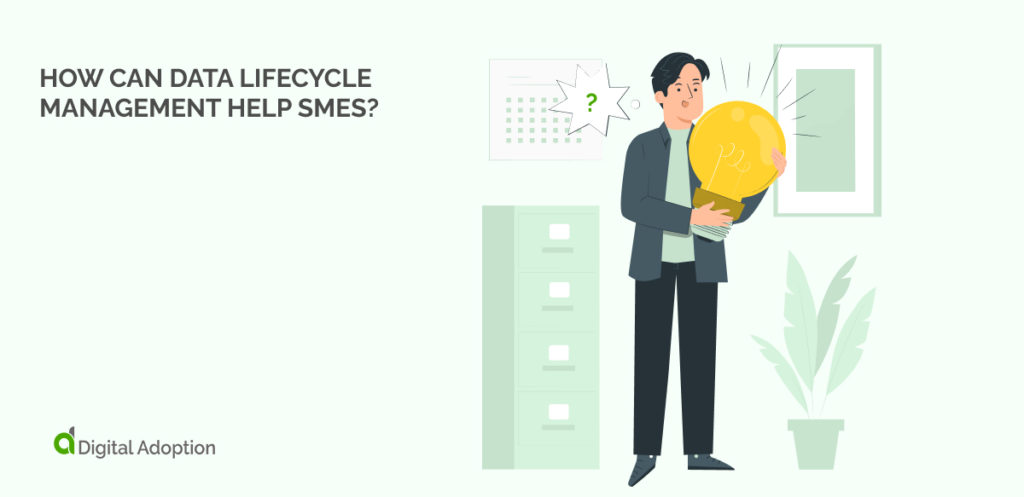
Relational databases, archives, and backups… might sound excessive for small to medium-sized enterprises (SMEs), but even small businesses can benefit from implementing data lifecycle management.
In a startup or small business environment where every resource has to stretch further, data can be your silver bullet for guaranteeing efficiency.
A data-driven approach will help you rest a little easier, knowing you’ve backed up your business decisions with high-quality data.
It also stands to reason that the consequences of poor data management are more severe for SMEs and startups.
Mishandling sensitive data can lead to fines but also compromises the trust customers have placed in your business— and that trust is virtually impossible to rebuild.
That said, you can follow the basic principles of data lifecycle management while making it easier for yourself and your business. For example:
- You could collect qualitative data using forms and surveys, which is much easier to manage and understand than quantitative usage data.
- You can use cloud-based solutions for storing your data, which are cost-effective, cheap, and low-hassle.
- There exists a category of tools known as Integration Platform as a Service (iPaaS) which allow you to more easily maintain and apply data, even if you’re not an expert.
- You don’t have to squeeze the maximum value out of your data. SMEs might benefit more from only using data to reap low-hanging fruit. This keeps time and effort to a minimum while still providing value.
What is Hot, Warm, and Cold Data?
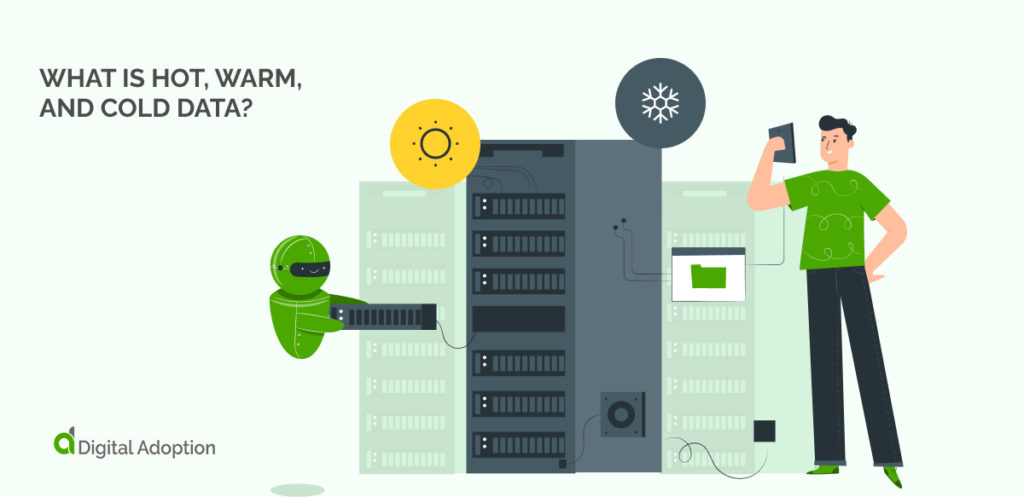
We use these temperature descriptors to categorize data based on how easily and quickly practitioners need access. This system helps businesses operate more efficiently by keeping the right data in the right place.
Hot Data
Hot data could also be called “active data.” It’s frequently accessed data that someone or something is currently working on. Analysts, practitioners, and software systems may require immediate access to it. For example, customer information during online transactions is hot data that your eCommerce system must be able to access quickly.
Another example of hot data is video files, which an editor stitches together.
Hot data is accessed frequently and is actively being used. You should store it in high-performance, low-capacity storage, close to the people or programs who want to use it, for example, on a local SSD drive.
Warm Data
Warm data is pertinent to some active projects that practitioners don’t need to access frequently. For example, if a business were launching a new product, it might keep all market research related to the product launch in warm storage.
Analysts need to access this data daily, but they move or copy it from warm storage to hot storage to work on it. Then move it back once they are done.
Warm storage is usually “capacity-optimized,”— meaning it’s kept on devices that allow a balance between capacity and performance. These might be local HDDs or production file servers.
Cold Data
You need to keep cold data, but people must actively work on it. You could consider it “inactive data.” Once a project manager finishes, they might move all the project data to cold storage.
Another example of cold data is financial, HR, or legal records, which you might have to keep just in case they’re needed.
The philosophy behind cold data is “cheap and deep.” In other words, high-capacity, low-performance devices like archives and file servers. The purpose of cold storage is to provide the cheapest means to keep data.
The Benefits Of Having A Set Process For Managing Data
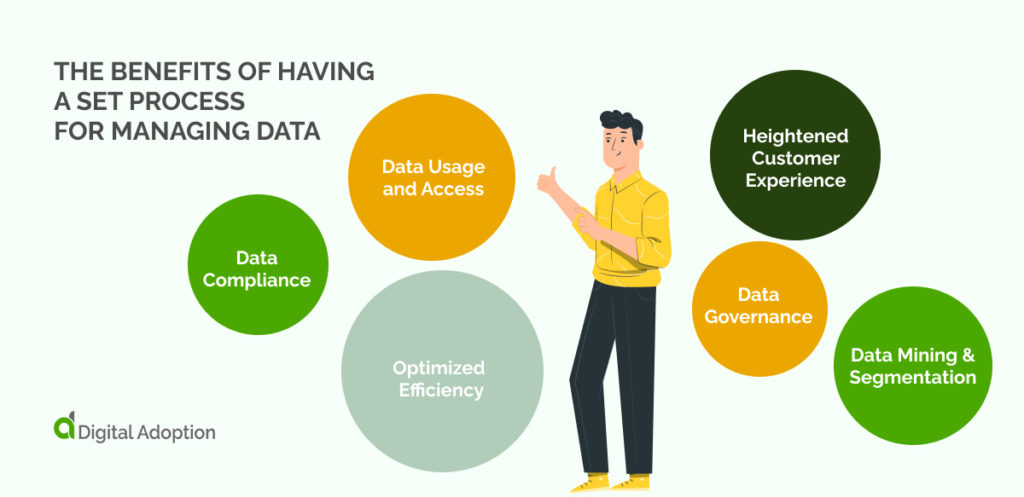
Data Compliance
For most of us, data compliance regulations are a real headache to wrap our heads around. Data lifecycle management can alleviate that headache because compliance considerations are built into the framework’s core, which helps you know when to keep and destroy data.
75% of countries have data localization rules, and companies with customers in different countries must adhere to them.
Collecting customer activity data and leveraging DLM to organize, store and protect them is the best way forward. Effective data lifecycle management allows businesses to easily access and analyze customer data to generate more insightful customer profiles.
Complying with these regulations independent of following a set process is daunting, risky, and probably not worth the work.
Data Usage and Access
Ensuring employees have quick access to relevant data enables them to work more agilely.
They spend less time trawling through data and more time creating value. They can make faster decisions and react to changes more quickly.
To put it briefly, it accelerates the time-to-value of any business initiative.
Optimized Efficiency
DLM allows you to “right-size” your data storage solutions, keeping costs low where possible and performance high where necessary.
Furthermore, a robust data-maintenance process helps develop a leaner database of more relevant data. Allowing you to trim the fat, so to speak, with regular data archiving.
Heightened Customer Experience
Well-managed data can give a business insight into a customer’s experience that wasn’t possible before—allowing analysts to marry traditional quantitative data with qualitative data to develop a deeper understanding of the customer journey.
This has even led to adoption of eXperience Level Agreements (XLAs) in some businesses, which are in place alongside Service Level Agreements (SLAs) to help measure and maintain the level of experience customers receive.
Data Governance
While data compliance refers to adherence to external regulations, data governance refers to internal standards.
An established process makes it easier for IT teams to govern data effectively and follow internal quality and security protocols. It’s a big help in data cleaning efforts to have transparent processes to follow.
Data Mining & Segmentation
Data mining and segmentation should be integral to any data lifecycle management strategy. Mining customer data allows a business to identify patterns and trends, which can then be used to refine customer segmentation for targeted marketing campaigns or product recommendations.
Data Lifecycle Management Best Practices
We’ve covered many of the factors that contribute to good data management. Like how and why you should have a process for data destruction, the importance of data encryption, and so on.
But data sharing isn’t just a bag of risks with no upside.
It’s time to talk about how businesses can get the most value out of data sharing and how to build an organization that uses data as a powerful tool for growth and agility.
Disseminated Data Management
Data management needs to be a company-wide effort. You should train all employees on handling data— at the very least, security and privacy protocols. Production teams and data analysts should be further trained to:
- Be able to access the data they need without having to raise requests with the IT
- Exercise good data-loss prevention (DLP)
- View data with a scrutinous eye, and assess whether they can move it to colder storage.
Knowing you’ve trained all employees in data management makes data sharing less risky.
Centralized Repository
Every business has security processes, and there’s no need to reinvent the wheel.
Keeping data in a centralized repository makes it more easily accessible for all employees while benefitting from internal security by default.
Remember: You should back up business data to a safe and secure environment to improve data redundancy.
Data Stewardship
Data stewardship helps keep an organization’s data quality high.
Assigning data stewards responsible for overseeing database management processes can be beneficial.
As is often the case with business processes, having a select few champions for data management in a business environment gives the rules more authority.
It also clarifies to users whom to reach out to with issues or questions. Data stewards aren’t solely responsible for administrative tasks like classifying data but can lend their expertise.
Data Training
Regulations continue to evolve, as do data management technologies. Continuous staff training ensures you meet regulatory changes with preparation and effectively utilize cutting-edge technology.
Additionally, data analysts should receive ongoing training to better categorize and manipulate data.
Data management is an iterative and ongoing process— there’s always room for improvement.
Data Aggregation
When analyzing data, it’s easy to get caught up in the details. Taking a step back and looking at the bigger picture is often beneficial.
Aggregating data can provide a high-level, macro view of a business’s data. This is just as useful for spotting trends and developing insights as the finer details are.
Data Standardization
Data standardization is trying to keep all data in the correct “types.” Data can be in the form of PDFs, survey results, or database entries.
Data of the same types are easier to marry or compare, which is a powerful tool.
You’ll get more out of collected data if you can keep it to the same file types.
Data Lifecycle Management Alternatives
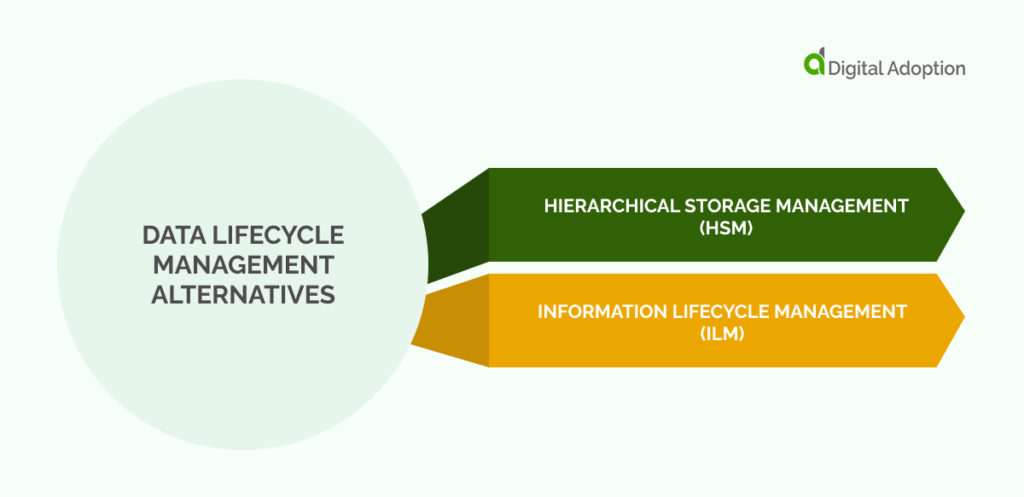
HSM— The Data Storage Supertool
Hierarchical Storage Management (HSM) is a tool that monitors data usage. HSM dynamically moves data to the appropriate storage system based on how often users access the data.
If data usage is high, it’s considered “active,” and HSM will move it to locations where retrieving data is quicker and easier.
HSM will move less critical data to colder storage media.
This takes a lot of the administration out of data management, as HSM does its job without business users knowing.
HSM takes a lot of the work out of deciding how to store data and can help with decisions around deleting data, as it’s easy to see how long data has been held without being accessed— this identifies suitable candidates for data destruction.
HSM isn’t so much an alternative to DLM as it is an additional option.
ILM
Information Lifecycle Management (ILM) is similar to DLM, with a few key advantages. Primarily, ILM also encompasses business processes on physically stored information.
Certificates, letters, contracts. Anything that isn’t digital. Information Lifecycle Management processes provide more in-depth guidance on how to manage data in this format and how it can be stored securely.
ILM also makes finding specific information related to a chosen topic easier. For example: Finding all of the data on one customer to dispose of it following a GDPR request.
DLM focuses more on categorization instead, and it’s easier to search my attributes like file type, size, or date. Which indexing function is more valuable is a matter of debate.
The bottom line is that ILM may be a better alternative if your business deals with digital and non-digital information. DLM is the more streamlined solution if you only deal with digital data.
Looking Ahead: What’s Next For Data Lifecycle Management?
The future of Data Lifecycle Management (DLM) is most certainly tied to the wider adoption of AI tools in businesses.
A 2021 McKinsey survey found that 57% of businesses have adopted AI in some form. And of those adoptees, 47% are using AI for some form of data analytics.
But businesses still haven’t realized the true power of AI-driven data. Another McKinsey report found that while 75% of companies in the metals and mining industry have implemented some form of AI, less than 15% have realized a meaningful impact.
There’s still a lot of work before AI becomes a must-have part of your data strategy.
DLM might also be affected by the Internet of Things (IoT) and edge computing. As IoT devices grow more powerful, they can process more data, which could change the way data is stored.
For example, hot or warm data could be stored on IoT-enabled devices, which can also process data— pushing data accessibility to new heights.
This, in turn, will affect a business’s Agile capabilities.
Under the Agile philosophy, analysts would be better positioned to make decisions based on the data they process. This leads to faster reactions to changes and lends a competitive edge to organizations that lean into it.
Finally, it’s essential to recognize that DLM must be iterative. Most importantly, for security purposes. New threats are constantly developing, and DLP technology, processes, and training must be continuously improved to stay ahead of malefactors.
The requirements of data management are only growing more critical and more complicated. Implementing a tried and tested set of practices like DLM is a proactive way to ensure you’re doing data right.