The prevalence of artificial intelligence (AI) has seen it leveraged for a near-infinite number of use cases.
The zeal to get ahead and position themselves as first-movers in the AI domain has also pushed businesses to look at the material risks AI poses. For one, the ability of AI to exhibit bias and generate potentially damaging outcomes.
AI uses machine learning (ML) models, natural language processing (NLP), data processing, and other technologies. If human bias (intentional or otherwise) enters any of these stages of AI development, AI outputs can become deeply distorted or misleading.
This article will explore the different types of AI bias that enterprises need to be aware of. This will help organizations identify, mitigate, and plan for future AI adoption and ensure ethical, accurate, and explainable solutions.
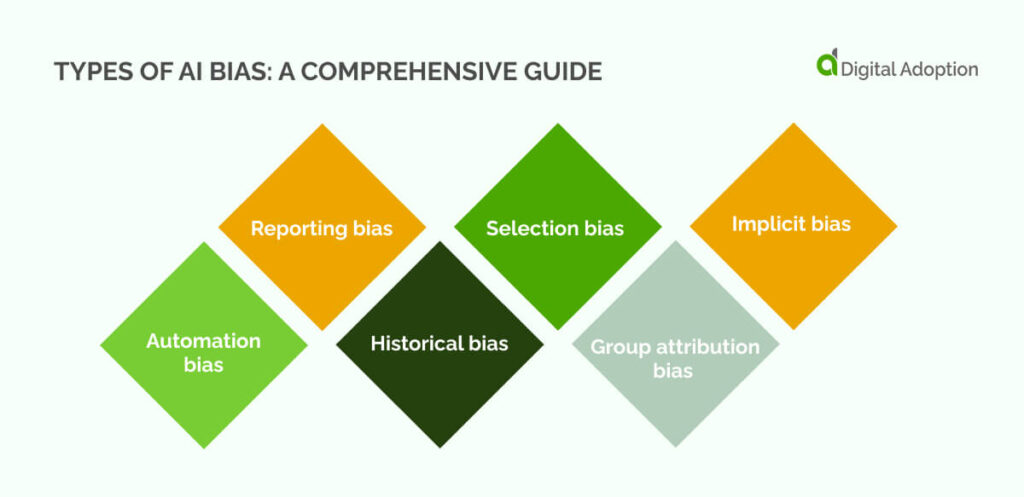
Automation bias
Automation bias is a type of AI bias that occurs when AI-automated outputs are favored over human-led recommendations.
The National Institute of Health (NIH) research states that preventable patient harm often results from multiple factors. Human error is among the most common root causes. Because of this, medical professionals may place heightened trust in AI systems to complete key actions and decision-making processes.
Over-reliance on AI systems perceived as trustworthy may lead users to accept AI-automated outputs without confirming the results or performing secondary evaluations. This bias can lead users to disregard non-automated actions, prioritizing AI-generated results even if system error rates occur.
If a patient from a marginalized group seeks care at a facility using biased AI, doctors may unknowingly provide inadequate advice. Automated recommendations can mirror prejudice or skewed reasoning from flawed training data or coding, resulting in medical guidance failing to meet patients’ needs.
As such, heightened skepticism of automation bias in AI must be maintained. AI in healthcare, if not properly managed, can lead to automation bias, resulting in misdiagnosis or incorrect treatments that may jeopardize patient safety and outcomes.
Reporting bias
Reporting bias is when the data used to train AI does not record enough real-world instances to reflect the frequency or nature of actual events. In reporting bias, the data underpinning AI outcomes is often steeped in reports, studies, and real-world evaluations.
Humans’ tendency to report on unusual or extraordinary events instead of everyday ‘mundane’ events can skew AI training data. The disproportionate understanding of extreme events can greatly imbalance model reasoning and leave AI without enough information to create a baseline for reality.
For example, if AI learns that December 25th is a significant religious holiday worldwide based on abundant data on Christmas, it may prioritize results centered on Christmas. If asked for a neutral overview of events for that day, reporting bias could lead AI to forget other important religious events that may also occur.
Historical bias
Historical bias is when the ML algorithms used to train AI contain historical datasets that reflect societal prejudices and biases. This causes bias to become baked into AI business models, where irrelevant and potentially damaging reasoning informs outputs and decision-making.
For AI-first enterprises, this type of AI bias can quickly turn seemingly innovative AI solutions into a significant ethical liability that has concrete impacts on people in the real world. For example, specific demographics and groups particularly vulnerable to prejudice in the past can be subjected to similar levels of egregiousness today if data isn’t vetted and up-to-date.
Banks using AI FinTech may be trained on outdated global compliance data, leading the system to follow obsolete regulations. This could result in inaccurate risk assessments, missed fraud warnings, or approvals that no longer meet legal standards. Over time, the AI may fail to adapt to new rules, exposing the bank to penalties and financial risks.
Selection bias
Selection bias occurs when datasets used to train AI models don’t accurately represent certain groups of people. This can lead to an inaccurate representation of reality, and the availability of chosen data can lead to misleading outcomes.
It’s an overarching type of AI bias with several different subtypes. It can take various forms, especially regarding how data is collected and which participants are chosen.
When certain groups are overrepresented or underrepresented in datasets, AI models may not accurately reflect the full diversity of behaviors, experiences, or demographics.
Let’s take a closer look at the different subtypes of selection bias:
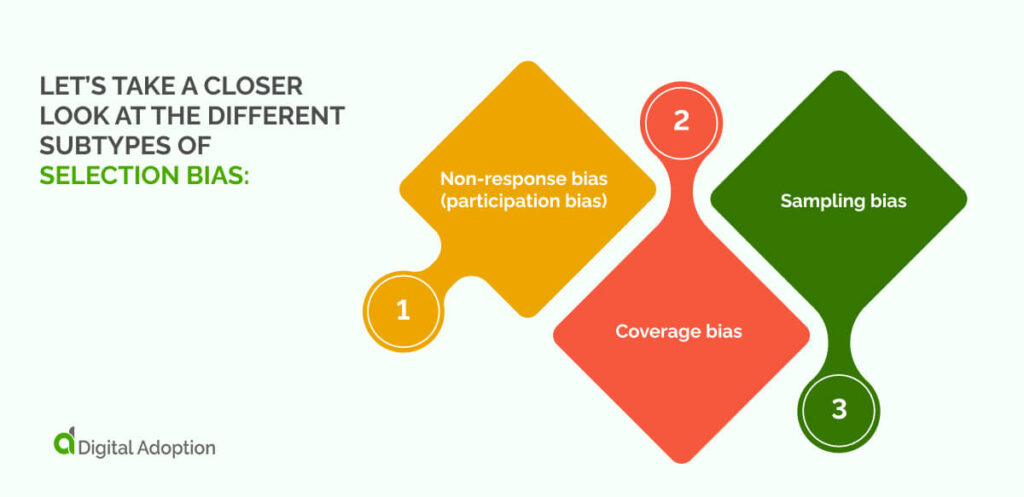
Non-response bias (participation bias)
Non-response bias occurs when certain groups don’t participate in data collection, causing an imbalance in who is represented. For example, if a survey about workplace wellness only gathers responses from happy employees, the AI may wrongly assume everyone is satisfied, leading to inaccurate conclusions about the broader population.
Coverage bias
Coverage bias happens when some groups are excluded from the dataset, affecting the AI’s predictions. If an AI system is trained exclusively on resumes from certain universities, it may overlook qualified candidates from less-represented schools. This can skew hiring decisions, favoring a narrower demographic and reducing diversity in the workforce.
Sampling bias
Sampling bias arises when the sample used to train AI doesn’t represent the larger population. If a facial recognition system is primarily trained on lighter-skinned individuals, it will struggle to recognize people with darker skin tones, leading to inaccurate results and reinforcing inequality.
Group attribution bias
Group attribution bias tends to make distinctions or assumptions about a particular group based on individuals’ generalized actions.
This type of AI bias assumes that the mutual actions of a specific group represent the behaviors, traits, or intentions of every individual within that group.
As a result, it can lead to unfair generalizations and reinforce stereotypes, impacting decision-making processes in AI systems.
Let’s take a closer look at the different subtypes of group attribution bias:
In-group bias
In-group bias happens when people favor their own group over others. This could be based on shared traits, like being in the same school, team, or community. People often give more trust, support, or advantages to their in-group members, even if there is no reason for it. This bias can lead to unfair treatment of others and strengthen divisions between different groups, affecting relationships and decision-making.
Out-group homogeneity bias
Out-group homogeneity bias occurs when people see members of other groups as being all the same. They assume everyone from a different group shares the same traits, behaviors, or characteristics. This bias can lead to stereotyping and misunderstandings because it ignores the diversity within out-groups. It can create negative perceptions and make it harder to relate to or empathize with people outside one’s group.
Implicit bias
Implicit bias is when people have unconscious beliefs or feelings about certain groups without realizing it.
These biases can make us treat people differently based on things like their race or gender, even if we don’t mean to. In AI, implicit bias can affect how computers make decisions.
If the data used to teach AI is based on these hidden biases, the AI might make unfair choices, like treating one group better. This can lead to problems like discrimination, where certain people are treated unfairly by the AI system.
Let’s take a closer look at the different subtypes of group implicit bias:
Experimenter’s bias (observer bias)
Experimenter’s bias happens when the person running an experiment influences the results without meaning. In AI, this can happen if the person testing the system shapes the data or how the results are shown based on their beliefs. For example, if an AI system is tested in a way that reflects the tester’s ideas, the AI may treat some groups unfairly, causing biased results.
Confirmation bias
Confirmation bias is when people only look for information that agrees with their beliefs. In AI, this can happen if the data used to train the system supports certain ideas while ignoring others. This can lead to AI systems that are unfair or inaccurate by reinforcing existing biases and treating some groups better than others.
How can enterprises identify AI bias?
AI bias is a serious issue that can lead to unfair outcomes and affect how people and businesses make decisions.
When AI systems are trained on biased data, they can make choices that harm certain groups, whether hiring, lending, or healthcare.
A proactive approach to AI bias also helps expand customer success and customer loyalty, as consumers are more likely to trust and stick with brands that prioritize fairness and inclusivity.
Actively checking for bias in AI systems means businesses ensure their products and services are fair, inclusive, and trustworthy.
This is especially important as physical and digital products continue shaping our future. When businesses work to reduce AI bias, they improve their products, build better relationships with customers, and contribute to a fairer society.
A thoughtful approach to AI bias will help enterprises stay competitive and innovative while creating a more equal world for everyone.
People Also Ask
-
How are organizations addressing AI bias?Organizations are working to reduce AI bias by using more diverse data and testing AI systems regularly to check for fairness. They also make sure different people are involved in creating AI to avoid unfair decisions. These steps help AI treat everyone fairly.
-
Can AI ever be completely free of bias?AI might never be completely free of bias because it learns from human data, which can be biased. But, by constantly improving AI and using diverse data, we can reduce bias and make AI fairer over time.
-
What are the harmful consequences of AI bias?AI bias can lead to unfair decisions, like discrimination in hiring or healthcare. It can hurt certain groups, limit opportunities, and create inequality. This can make people lose trust in AI and hurt businesses that rely on it.