Generative AI and machine learning can be two sides of the same coin if you know how to utilize them.
Both technologies are leading to the creation of new roles. In 2022, the top-performing organizations utilizing AI were twice as likely to hire machine learning engineers. The explosion in the popularity of generative AI applications has introduced roles like AI prompt engineers and deep learning architects, which enterprises are beginning to invest in.
Introducing new roles can effectively leverage your knowledge of how generative AI and machine learning can work together to achieve a successful AI-driven digital transformation.
This article will begin by providing definitions of generative AI and machine learning. We will then move on to the differences between these technologies and AI examples of how they work together.
After reading, you will rise above competitors as you learn to utilize generative AI and machine learning for higher efficiency and revenue.
What is generative AI?
Generative AI uses large amounts of data to cheaply and quickly generate high-quality paragraphs, images, and music. Organizations use it to answer customer support queries, create advertising images, and drive email outreach.
ChatGPT by OpenAI, Gemini by Google (previously Bard), and Copilot by Microsoft are the three most popular generative AI applications.
What is machine learning (ML)?
Machine learning (ML) is a branch of artificial intelligence (AI) that uses data and algorithms to enable AI to mimic human learning and progressively enhance its accuracy.
Machine learning is essential in many aspects of sales and marketing. Organizations use it to successfully recommend products through social media algorithms, email automation, and predictive analytics.
What is the difference between generative AI and machine learning?
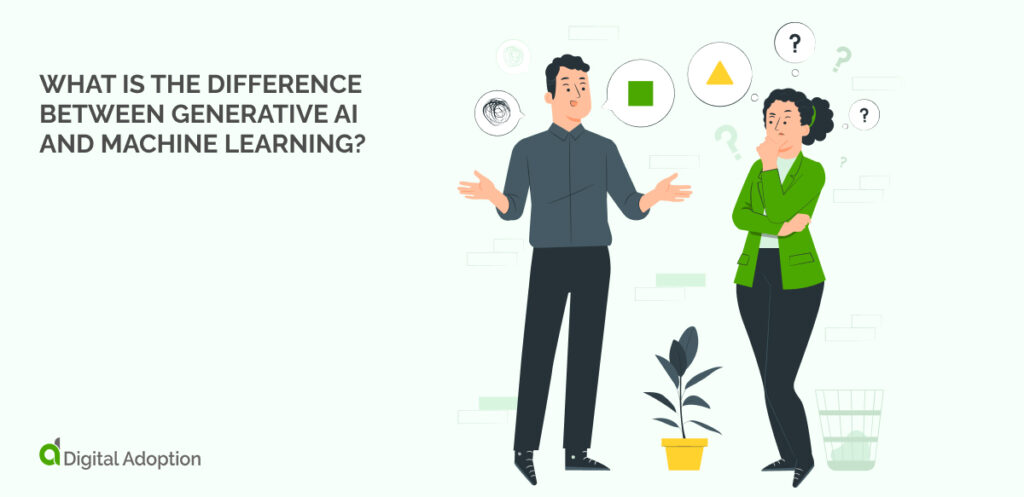
While generative AI and machine learning are branches of AI, they serve distinct functions and applications that fit different AI business models.
Machine learning excels at making predictions by identifying patterns in data, whereas generative AI focuses on creating new, original content from learned data patterns. Each has its value and caters to different business needs.
In business contexts, machine learning aids in predictive analytics, fraud detection, and process automation. Conversely, generative AI is beneficial in areas requiring creative output, such as personalized customer experiences, product development, and content creation.
The differences between these two AI types become clearer when we view them in a comparison attributes table.
Parameters | Generative AI | Machine learning |
Output type | Generative AI can create images, music, video, code, and content besides text. | Machine learning output includes predictions, classifications, and insights derived from analyzing data patterns to inform decisions and automate processes. |
Data availability | Generative AI requires diverse datasets for different types of content. | Data availability influences machine learning performance; adequate, diverse data facilitates accurate model training, while limited data may impede effectiveness. |
Task complexity | Generative AI suits complex, creative content generation and tasks requiring diverse outputs. | Task complexity varies for machine learning, based on individual task complexity for a process. |
Resources and model size | Larger generative AI models need massive computational resources and storage. | ML models usually need less computational power. Once they have received training, they can efficiently handle large datasets |
Training data type | High-quality, diverse training data is crucial for generative AI to generate meaningful and creative outputs. | ML depends on numerical or categorical data. |
Industry use case domain | Creative fields.Marketing.Advertising.Content creation. | Product recommendations.Email automation and spam filtering.Financial accuracy.Social media optimization. |
Developmental expertise | Developing and fine-tuning generative AI models can be complex and requires expertise in machine learning and domain-specific knowledge. | Machine learning uses a lot of complex mathematical concepts like calculus, probability, linear algebra, and statistics. These needs make it challenging to develop new ML models. |
Ethics and privacy | Generative AI outputs are subjective. Political biasNarrow researchFactual inaccuracies.Replacing human employees. | Data breaches can lead to financial fraud. |
Combining generative AI and machine learning offers numerous benefits, such as enhanced creativity and predictive accuracy. Generative AI can create innovative content while machine learning optimizes and personalizes it based on user data.
For example, an enterprise can use generative AI to produce personalized marketing materials, while machine learning algorithms analyze customer responses to refine and target future campaigns.
This enhances customer engagement, drives sales, and promotes more efficient and effective marketing strategies.
Consider the differences between generative AI and machine learning, apply each to the most appropriate use case, and combine them correctly. This approach will ensure that your investment will deliver ROI.
Examples of how generative AI and machine learning work together
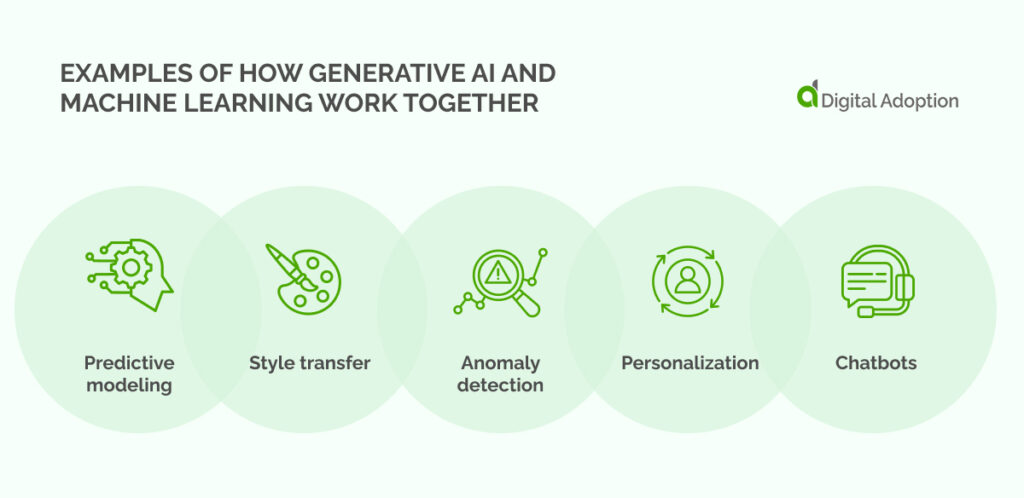
Generative AI and machine learning can complement each other, offering integrated AI solutions to diverse challenges. AI adoption rates are currently negatively impacted by concerns by organizations about becoming dependent on it.
Despite 43% of companies being concerned about AI dependence, understanding examples of how generative AI and machine learning work together may encourage companies to realize that AI can improve processes alongside human input.
This approach enhances data-driven predictions. It can also support content personalization for individual customers or groups, detect anomalies, and efficiently create innovative, targeted solutions.
Predictive modeling
In predictive modeling, generative AI simulates various future scenarios. The result is a rich dataset for machine learning algorithms that various enterprises use to anticipate outcomes and make informed decisions.
One example is how generative AI creates potential market conditions or customer behaviors. This data allows machine learning models to predict future trends more accurately. This approach enhances risk management, resource allocation, and strategic planning by considering various possibilities.
The synergy between generative AI and machine learning ensures that predictions are data-driven and account for complex, dynamic variables, providing enterprises with a robust forecasting tool.
Style transfer
Generative AI creates unique styles for images or texts in many distinct personalities or voices, which machine learning algorithms apply to various content.
For example, generative AI can design artistic styles in the media industry, and machine learning can apply these styles to videos or articles.
This approach maintains consistency and quality for consumers. The technique enables enterprises to efficiently produce visually appealing and customized content to keep customers engaged and loyal to their brand.
In marketing, style transfer can personalize advertising materials to match brand aesthetics. The collaboration between generative AI and machine learning helps enterprises quickly adapt to changing trends and preferences, enhancing their content creation capabilities and audience engagement.
Anomaly detection
Generative AI models simulate normal operational behavior, creating a baseline for machine learning algorithms to identify anomalies. This method is crucial for industries like cybersecurity, where detecting unusual patterns early can prevent breaches.
Machine learning systems can more accurately flag deviations by learning typical behavior from generative models. This action reduces false positives and enhances the detection of genuinely suspicious activities.
Enterprises benefit from improved security measures, reduced downtime, and prevented potential losses. Integrating generative AI and machine learning creates a proactive approach to anomaly detection, safeguarding enterprise systems and data.
Personalization
Machine learning tailors generative AI outputs to match user preferences. This combination enhances personalization in customer interactions.
For instance, generative AI can make personalized product recommendations, ads, or content. These utilize user data that organizations get from social media.
Machine learning algorithms analyze user behavior and preferences. They use the data to fine-tune these outputs, ensuring they are relevant and engaging. In e-commerce, this leads to higher conversion rates and customer satisfaction.
Marketing and sales means delivering more engaging material. The department must target content to lead to sales. AI is creative, and machine learning is powerful. Combining them lets enterprises give personalized experiences. This approach drives customer loyalty and business growth.
Chatbots
Generative AI and machine learning combine. They create advanced chatbots. The bots enhance customer interaction for enterprises.
Generative AI, like ChatGPT, makes human-like responses. This feature lets chatbots engage users naturally and contextually. However, machine learning analyzes user data to improve chatbot performance. It learns from interactions and uses them to personalize responses and predict user needs.
This teamwork lets chatbots handle many queries. They provide accurate information and increase user satisfaction. Businesses benefit from automating customer support. Benefits include lower costs and a seamless, personalized user experience. It strengthens customer relationships and drives business growth.
These examples show the strong link between machine learning and generative AI and the high value of these technologies in many businesses. Success depends on understanding these always-changing technologies. You must deploy them to use their strengths well and enjoy their full power.
Combine generative AI and machine learning for long-term benefits
Generative AI is booming today. It helps firms be more efficient and do higher-level tasks. Machine learning can add to generative AI in many ways. It can aid with chatbots, personalization, and predictive modeling.
When you combine these tools, you can enjoy several benefits. Using machines to analyze large datasets brings the first benefits you’ll notice. They make realistic data. This approach improves model training and performance.
Another benefit is higher accuracy. Use machine learning to predict. Use generative AI to simulate. This process improves your decision accuracy.
However, the biggest benefit of this combination is personalization. It works across many uses. Machine learning tailors AI outputs to your preferences, providing customized user experiences. The combination increases sales and customer satisfaction.
Using generative AI with machine learning improves customer relationships, grows revenue, allows you to invest in more innovations, and secures your organization’s future.